Dataset:
During pretraining, we use “high-level” ground truth texts from the HumanML3D dataset and “low-level” ground truth text labels from the BABEL dataset.
HumanML3D
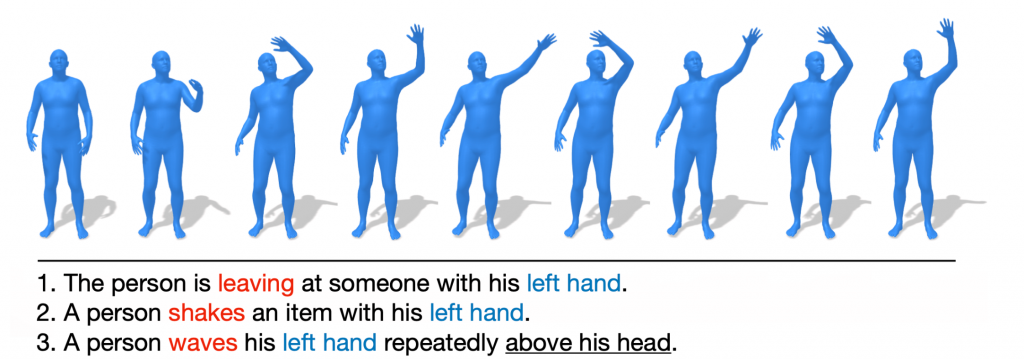
- Statistics: 14,616 motions and 44,970 descriptions composed by 5,371 distinct words. The total length of motions amounts to 28.59 hours, corresponding to the “high-level” conditioning in our model
BABEL
- Contains 28k sequences with per-frame action label, for a total of 63k frame labels, corresponding to the “low-level” conditioning in our model
- Over 250 unique action categories
Quantitative Result
Qualitative Result
Comparison with Baseline Model
Text Prompt: “a man does a push-up and then uses his arms to balance himself back to his feet”
Our Result:
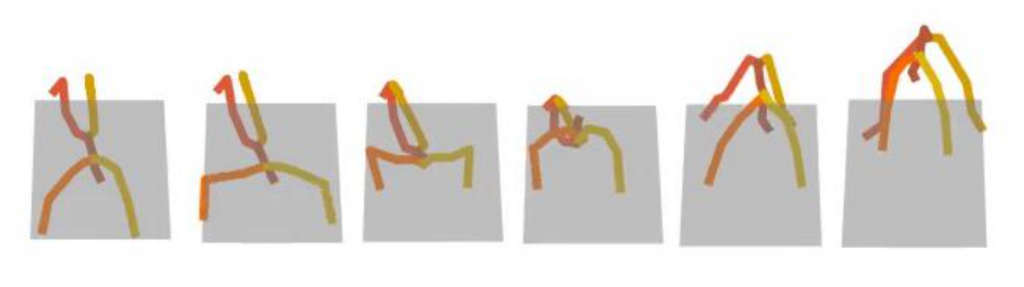
Baseline:
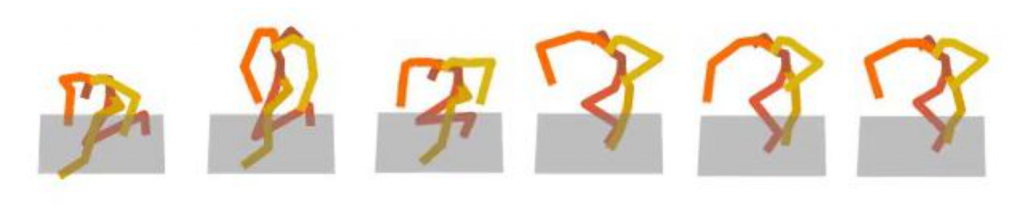
Here the text prompt is unseen in the training set, and has some complexity. It can be observed that baseline method can not generate motion of “push-up” correctly. However, with Large Language Model to further explain “push-up” and “balance himself” to the model, our frameworks successfully generates the correct “push-up” motion and the subsequent motions.
Motion Synthesis with Same High-Level, Different Low-Level Text Prompts
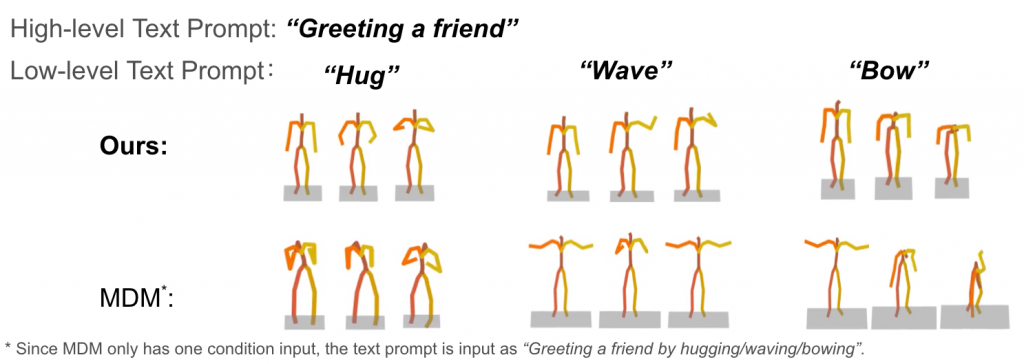
Additional Qualitative Results
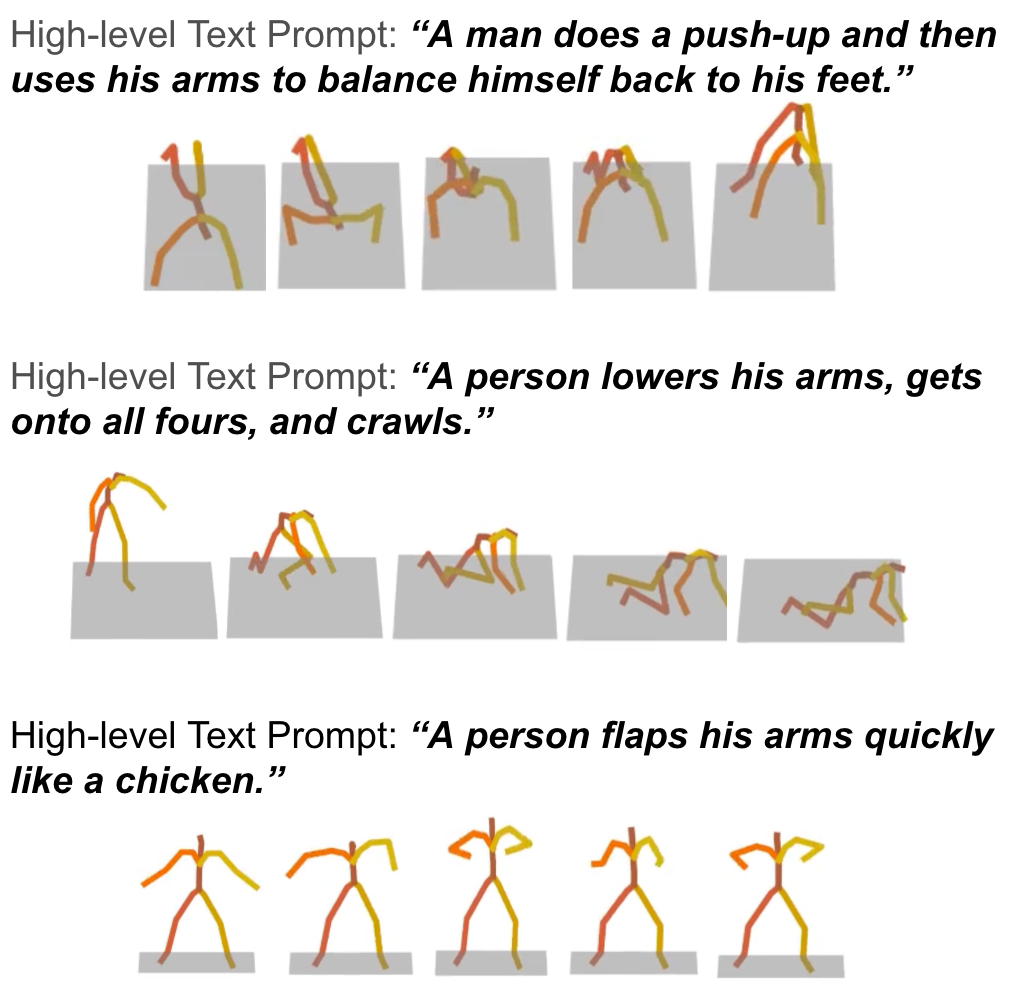
GPT-3 Prompting Examples
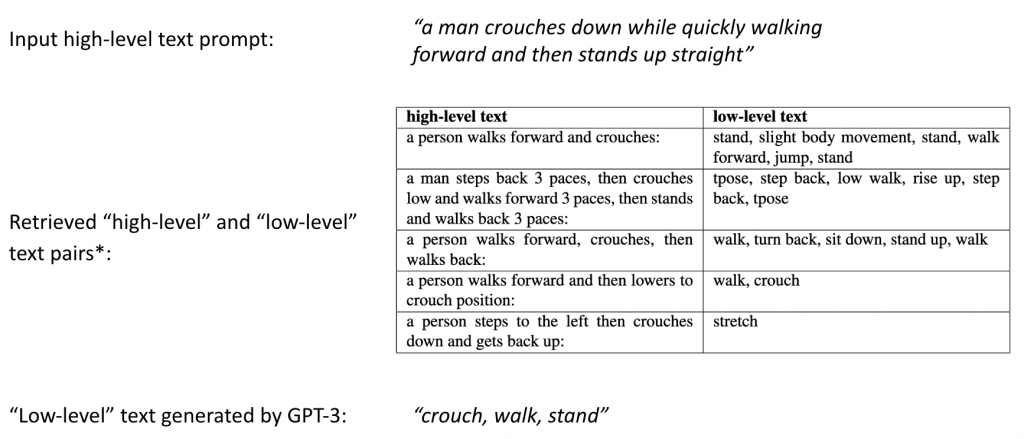