SRT
Our current work is inspired by Scene representation transformers which use data-driven priors to generate novel views from multi-view inputs. Given a set of multiview inputs and a query pose, the SRT generates the image corresponding to the query pose.
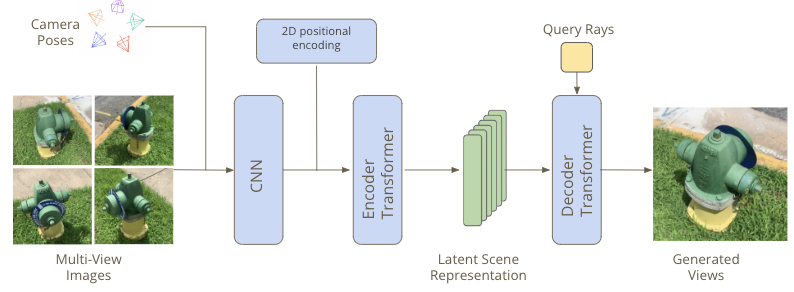
Our Baseline model shares a significant resemblance with SRT, except for two distinguishing factors. Firstly, we encode pose features to the image features instead of encoding them directly to the images. This approach allows us to utilize geometric priors to train the transformer. Secondly, unlike the conventional approach of using query pose to generate a novel view, we use query image features to predict the pose.
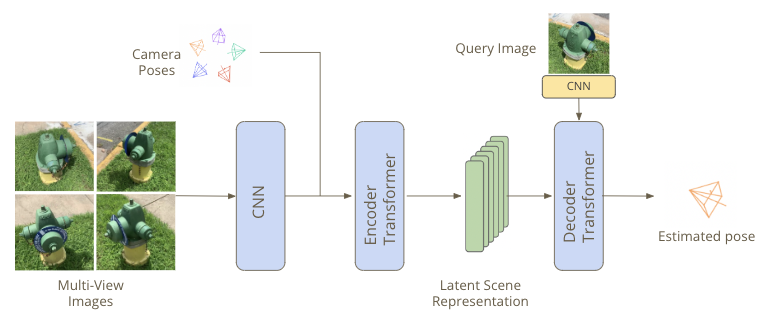
Results
We trained with only three input images. Below are some results from the baseline experiment.
Qualitative Results
Given three input images with camera views and one query image, our model can regress the pose of the query image. Notice that the query image’s baseline is huge, yet the model can accurately regress the pose.
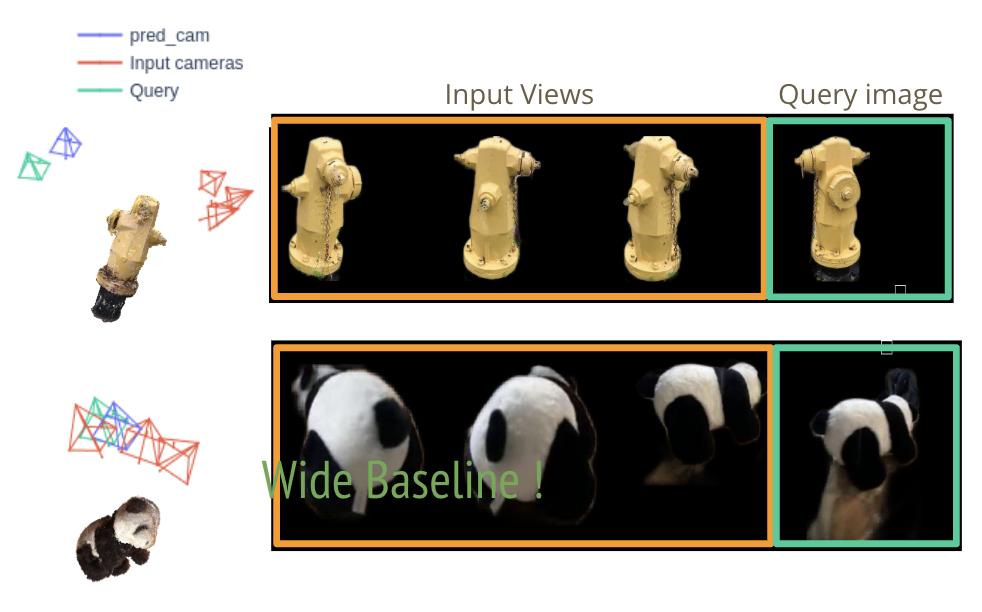
Quantitative Results
After evaluating our model to a subset of the Co3D v2 dataset, we established that it performs remarkably well, achieving an accuracy of approximately 85% despite using only three input views.
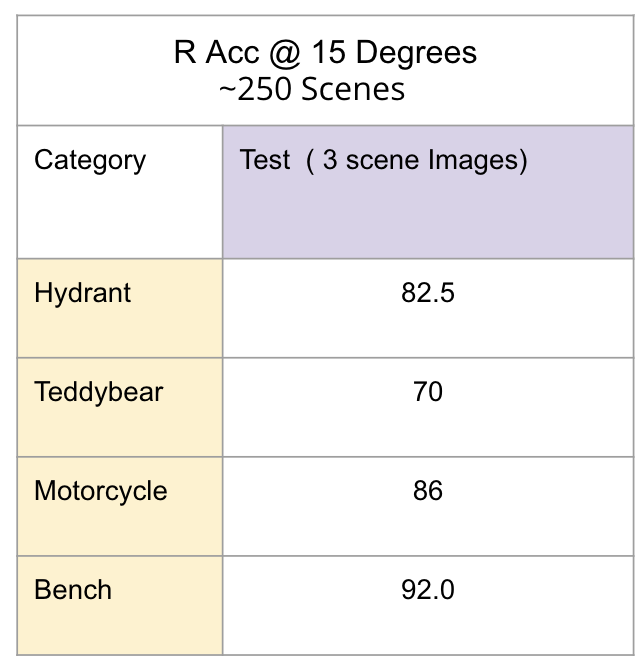