Comparison with Baselines
We compare our reconstructions with 2 template-free baselines iHOI and HHOR. iHOI makes per frame prediction while HHOR reconstructs 3D from video clips without any data driven prior. We observe that our method performs much better in terms of visual quality as well as F1 scores and Chamfer Distance metrics.

Table: Comparing F@5mm, F@10mm and CD scores for object reconstruction on HOI4D
a. HOI in image frame (top left)
b. HOI in a novel view (top right)
c. HOI at middle time step (bottom left)
d. Rigid object reconstruction (bottom right)
Left to right: GT | Ours | IHOI | HHOR
Ablation: How does each prior help?
We train separate diffusion models that only condition on one of the priors: category/ hand. We observe that category prior ensures better object reconstructions while hand prior gives us better hand-object relations.
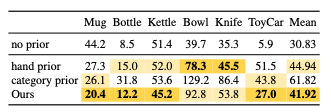
Table: Analysis of Category and hand prior on HOI Reconstruction: Comparing chamfer distance in hand frame (HOI4D dataset). Note: (no prior) doesn’t generate realistic shapes
Left to right: GT | Ours | Category Prior | Hand Prior | No prior
Ablation: Which geometry modality matters?
We analyse modality contribution when distilling to 3D shapes by setting their weight in SDS loss to 0. We observe that Normal > Depth ~= Mask (crucial order). Depth modality has also shown to ensure better hand object alignment.
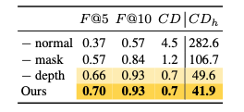
Table: Ablation without surface normal, mask and depth: Comparing F1 scores (5,10mm) and chamfer distance in object frame for object reconstruction error and comparing CD in hand frame for hand object alignment
Left to right: GT | Ours | w/o Depth | w/o Mask | w/o Normal