We all, in general, not only run and work, but also interact with the object around us. Like playing tennis, drinking tea etc. Instead of just reconstructing the human and the hand, we also want to reconstruct the racket and tennis ball that the player holds, the mouse and pen in someone’s hands. In particular, we need to understand the object in hand and the type of interaction between the hand and the object. In this project, we focus on hand-object reconstruction. This is a very challenging problem because hands and objects often suffer from heavy mutual-occlusion, so reconstructing them could be quite difficult.
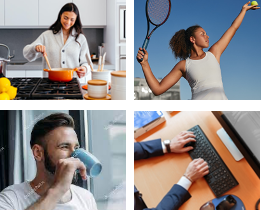
Imagine watching a video of human cooking in the kitchen. We humans can easily understand the underlying 3D hand-object-interactions in the physical world, such as contact regions, 3D relations between hands and objects. More impressively, we are able to hallucinate the 3D shape even if we have not seen some views of the object, such as the bottom part of a cooking pan.
In this work, we aim to reconstruct 3D hand-object interactions (HOI) from a monocular video. Prior works of HOI from videos typically assume known object templates and jointly fit hand and object pose to the 3D instance. In contrast, we aim to reconstruct unknown generic objects. To do so, on one hand, many works have studied 3D reconstruction for generic scenes by optimizing a neural field to match the given image collections or video. However, they typically require all object regions visible in some of the frames. On the other hand, recent works have studied a data-driven approach to learn hand-object prior but they do not consider temporal consistency in videos. In this work, we propose to leverage progress from both directions to reconstruct the hand and object from Internet video clips. We first explore reconstruction for hand-held rigid object and then try to extend the architecture to articulating objects.