Rohan Chacko
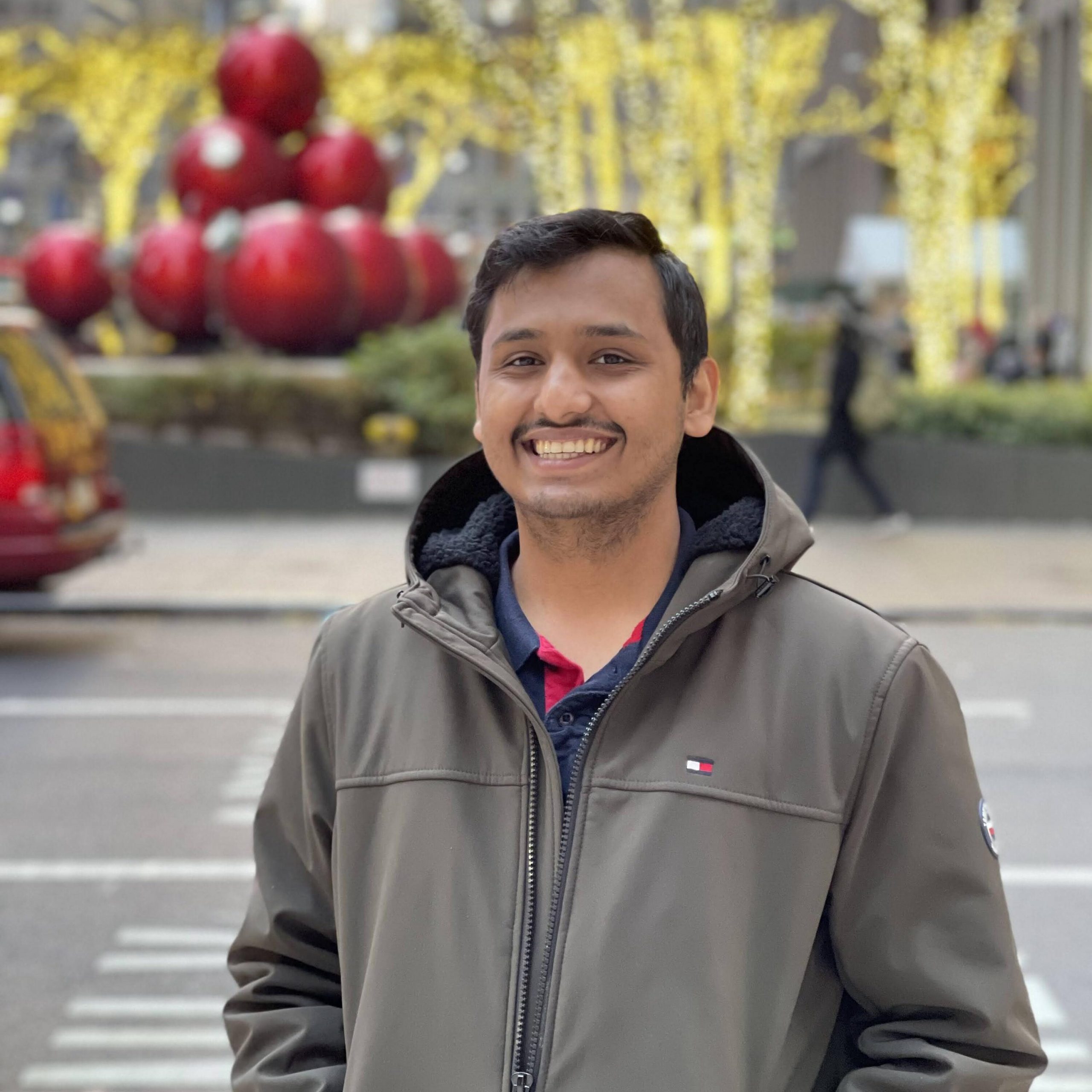
Rohan is a graduate student at Carnegie Mellon University pursuing his master’s in Computer Vision. Previously, he was an undergraduate at the International Institute of Information Technology, Hyderabad, India. Over there, he worked on single-view methods for 3D human reconstruction under the guidance of Dr. P.J. Narayanan and Dr. Avinash Sharma. His work primarily involved formulating a novel shape representation for efficient high-quality 3D renderings of humans under loose clothing. He has also interned at Dr. David Held‘s lab working on zero-shot object segmentation methods. His interests are broadly in the field of 3D vision and learning.
Sri Nitchith Akula
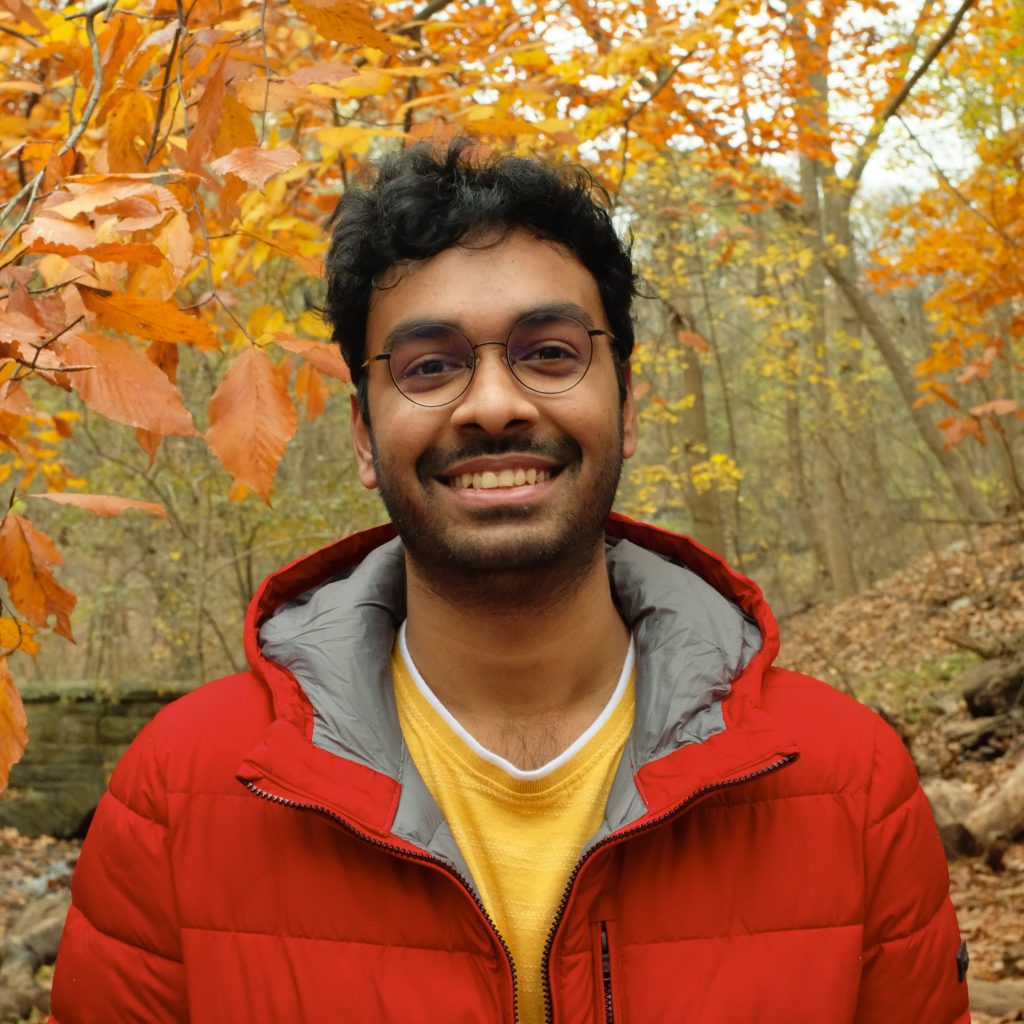
Sri Nitchith is a graduate student at Carnegie Mellon University pursuing his master’s in Computer Vision. Previously, he worked at Samsung R&D Institute India – Bangalore from 2016 – 2020. His work primarily involved improving video compression and image super-resolution using Deep Learning for mobile devices. He completed his undergraduate at the Indian Institute of Technology, Bombay in 2016. During that time, he worked with Prof. Bipin Rajendran on Neuromorphic Computing.
Dr. Aswin Sankaranarayanan
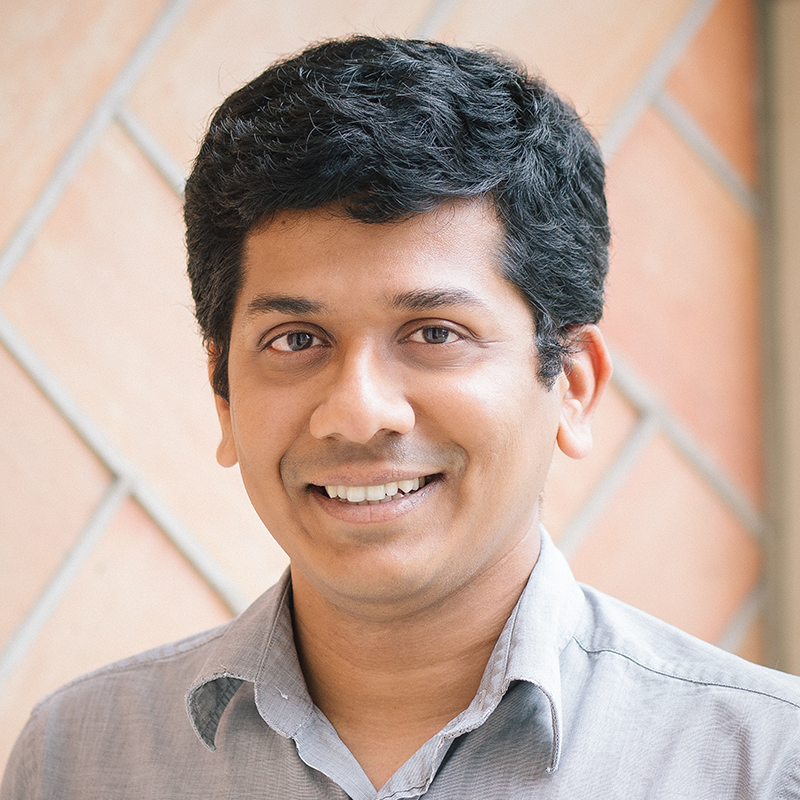
Aswin’s research deals with understanding the interaction of light with materials, devising theories and imaging architectures to capture these interactions, and finally developing a deeper understanding of the world around us based on these interactions. While these interactions involve very high-dimensional signals, there are underlying structures that enable them to be modeled parsimoniously using low-dimensional models. Aswin’s research identifies low-dimensional models for high-dimensional visual signals using both physics-based and learning-based formulates, and develop imaging architectures and algorithms that exploit these low-dimensional models for efficient sensing and inference.
Project Responsibilities
In Spring ’22, we worked on the literature survey and brainstormed ideas to experiment. As part of this, we created our own synthetic Focus-Aperture stack dataset, implemented the MPI-based approach, and concluded that it was advantageous to focus on a NeRF-based approach. We took inspiration from Mip-NeRF to model defocused images. We conducted some early experiments that did not yield satisfactory results.
In Fall’22, we identified issues in our dataset and implementation. We fixed all those issues and conducted all the experiments shown in the NeRF approach. It’s interesting to see that a model trained on defocused images can generate all-in-focus images and different defocus effects during test time.