TL;DR
OVERVIEW VIDEO: https://www.youtube.com/watch?v=e_dl2HbUTRY
Task: We study the task of synthesizing novel views of an object given a few (2-6) input images with associated camera poses.
Method: Our work is inspired by recent ‘geometry-free’ approaches wherein multi-view images are encoded as a (global) set-latent representation, which is then used to decode novel viewpoints. While this representation yields (coarsely) accurate renderings, the lack of geometric reasoning limits the quality of these outputs. To overcome this limitation, we propose ‘Geometry-biased Transformers’ (GBTs) that incorporate geometric inductive biases in the set-latent representation-based inference to encourage multi-view geometric consistency.
Results: We validate our approach on the real-world CO3D dataset, where we train our system over 10 categories and evaluate its view-synthesis ability for novel objects as well as unseen categories. We empirically validate the benefits of the proposed geometric biases and show that our approach significantly improves over prior works.
Motivation
Novel View Synthesis. The ability to infer the 3D structure of the world is crucial for a wide range of applications e.g. robotic agents that can manipulate generic objects, AR assistants that can understand user activities, or even mapping and exploration techniques. While we humans are able to intuitively reason about the structure of the world around us, it is an open research challenge to build autonomous agents with a matching capability. One such task where humans excel is hallucinating what an object looks like from a partial observation, which demonstrates a rich 3D understanding of the world.
Sparse-view 3D Reconstruction. In particular, we are interested in the task of Novel View Synthesis from sparse observations. Given just a few images depicting an object, we humans can easily imagine its appearance from novel viewpoints. For instance, consider the first image of the hydrant shown below and imagine rotating it clockwise.
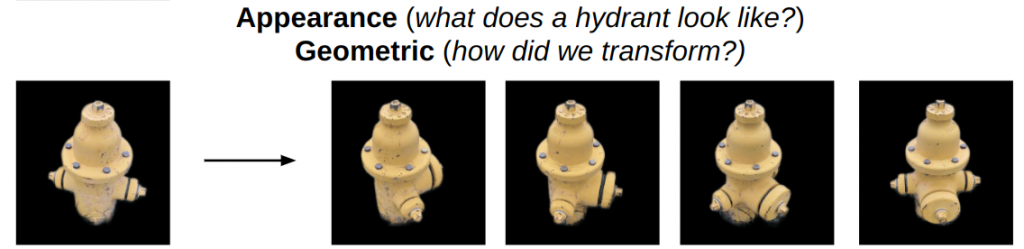
We intuitively understand that this would move the small outlet towards the front and right. We can also imagine rotating the hydrant further and know that the (currently occluded) central outlet will eventually become visible on the right. These examples serve to highlight that this task of novel-view synthesis requires an understanding about both the geometric transformations e.g. motion of the visible surfaces, as well as the appearance e.g. occlusions and symmetries to allow for realistic extrapolations. While prior works only partially capture the above aspects, we develop an approach that incorporates reasoning along both these aspects to render accurate novel views of a previously unseen object.
Problem Setup
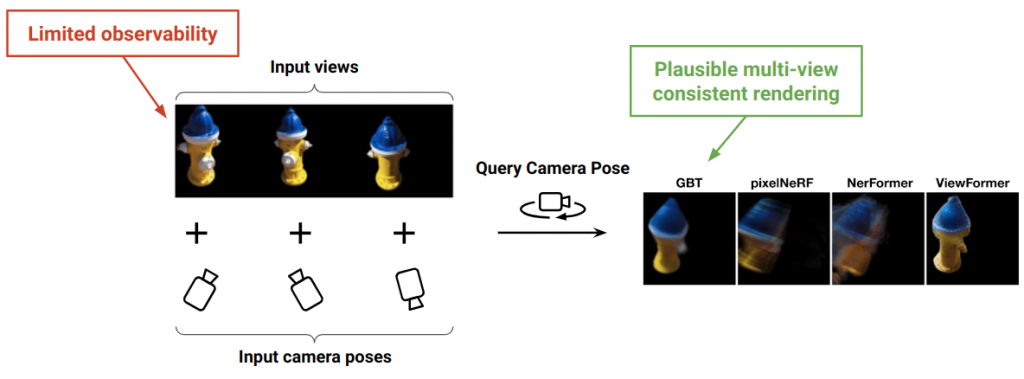
Novel-view Synthesis from sparse observations. Given a few (2-6) images of an unseen object with associated camera poses, we wish to render the object from arbitrary viewpoints. This is a challenging task because the sparse input views only contain limited information about the appearance and the geometry of the object. The overarching objective is to faithfully reconstruct what is observed and plausibly hallucinate that which is hidden or unseen.
In this constrained setting, our proposed approach GBT is able to synthesize realistic multi-view consistent results. Note, while we describe an approach for objects, it can be extended to images of arbitrary scenes as well.
Contributions
We present several geometrically-principled modifications to the transformer layers to suit the task of 3D reasoning.
We show the benefits and limitations of the contemporary state-of-the-art methods, and describe several design choices aimed to bridge the gap in the literature.
Our method achieves superior results compared to the state-of-the-art methods.