Benchmark Results
We compared our method to published state-of-the-art methods on standard benchmarks for 3D and 4D lidar panoptic segmentation on Panoptic nuScenes and SemanticKITTI.
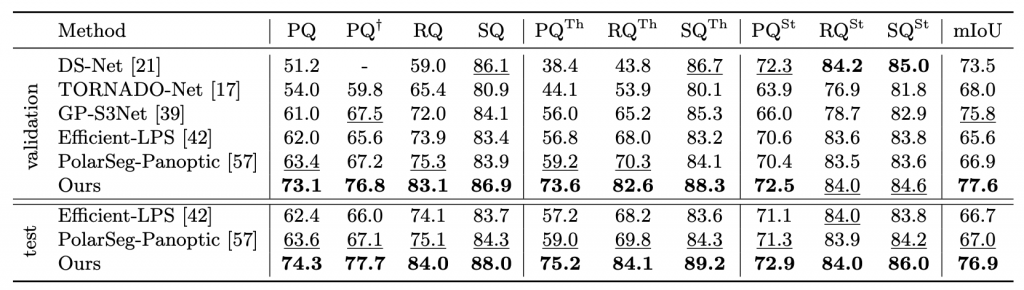
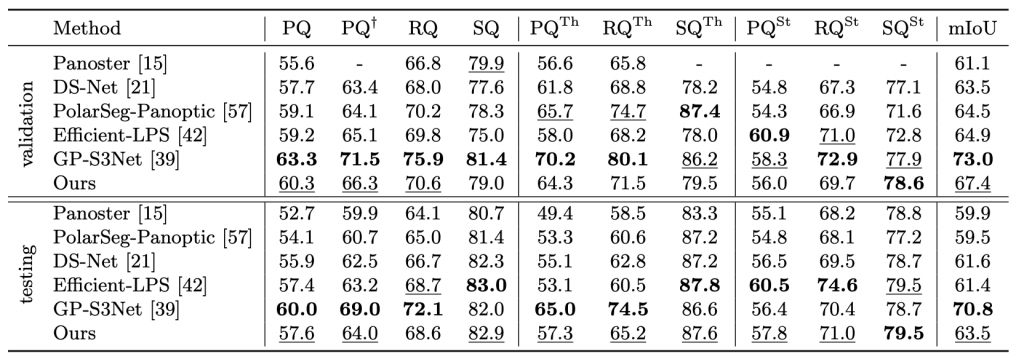
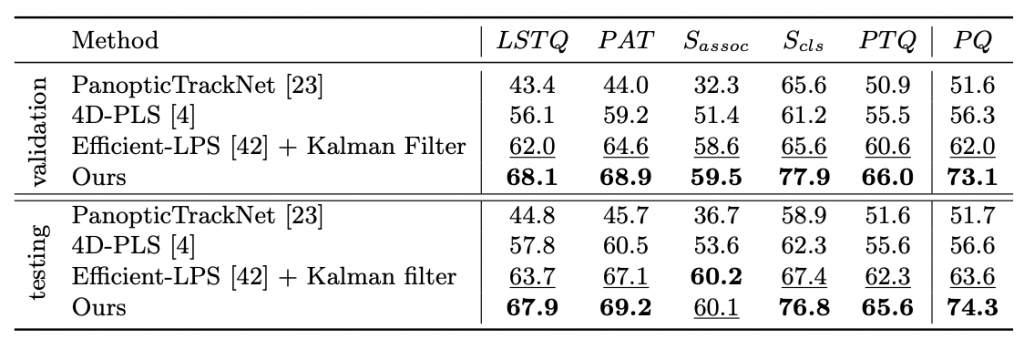
Ablation Studies
Modal recognition or center-offset regression?

All methods are sharing same semantic segmentation network. The first two entries used center offsets followed by clustering to obtain instances, while the third entry would use our modal detector followed by PointSegMLP to obtain instances. As the semantic segmentation networks are identical, this experiment highlights the effectiveness of our modal detection branch.
Separate models or a unified model?

First entry has two separate models for semantic segmentation & modal instance recognition. we train a single network for semantic segmentation and modal instance recognition and segmentation (i.e. our full model), this yields overall best results with a PQ score of 73.1. This indicates co-learning helps communication between two tasks and benefit the final performance.
Can our model benefit from amodal labels?

In this experiment, we evaluate the impact of modal training on our detection component. While our network trained with modal labels is already state-of-the-art, this experiment confirms we can further benefit from amodal recognition whenever such labels are available. Extra information (e.g. orientation, full extent) is available in amodal labels, which may help.
PointSegMLP?
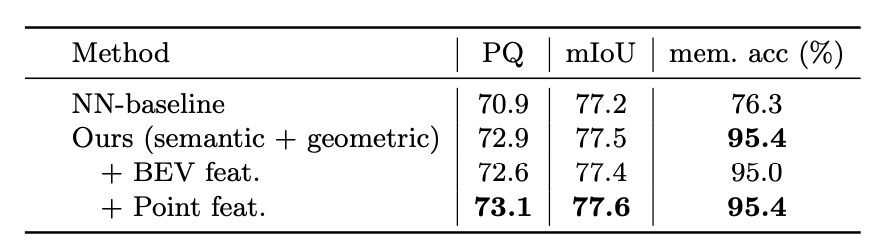
we compare our PointSegMLP with a simple, yet surprisingly effective nearest neighbor heuristic (NN-baseline). As can be seen, our learning-based PointSegMLP based on 3D positions and semantic predictions already significantly outperforms the heuristic. While instance (BEV) features in isolation do not benefit our model, they further improve the performance when combined with per-point semantic features.
Qualitative Results
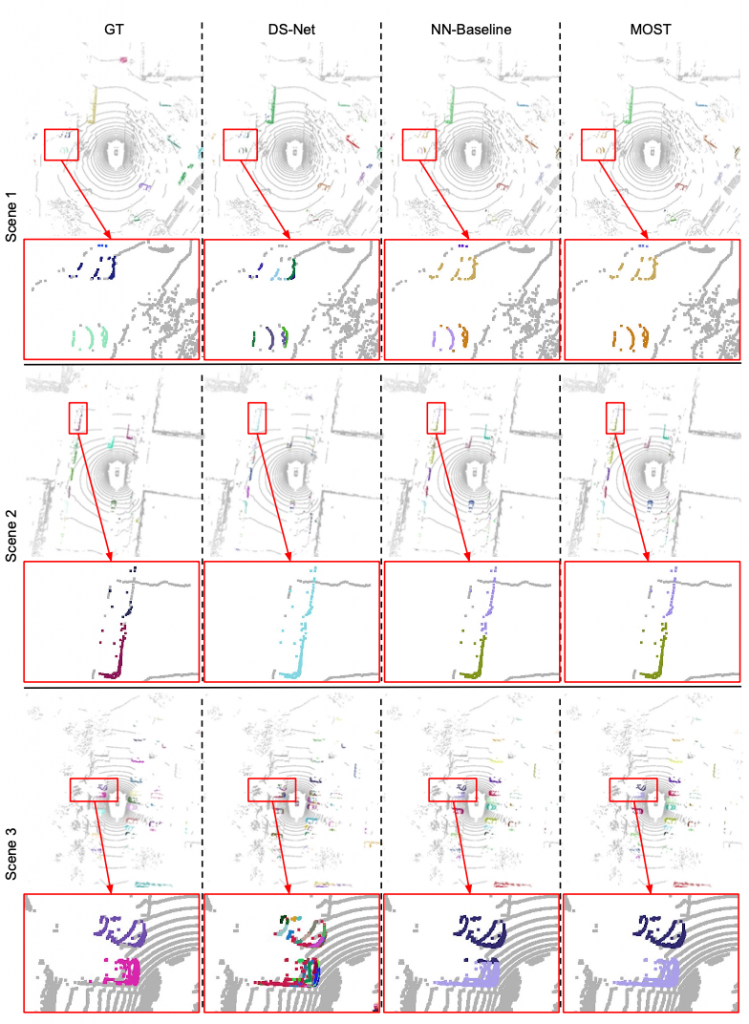
Figure 1. Qualitative comparison of DS-Net, our NN-Baseline and MOST
More qualitative results can be accessed from here.