Overview: How to Achieve Amodal Perception?
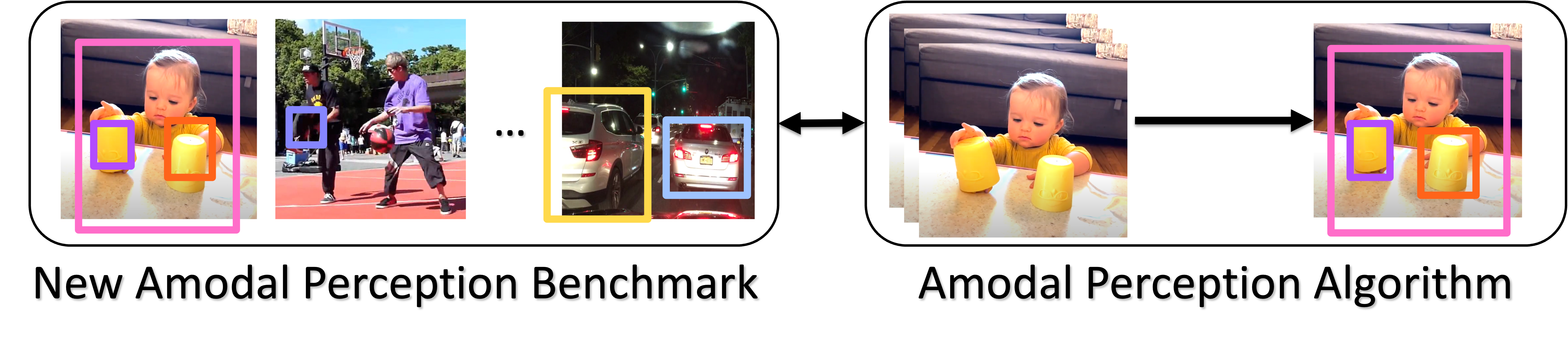
We created the largest real-world amodal perception benchmark and a light-weight plug-in module that could transform any existing trackers into amodal ones with limited training data.
TAO-Amodal: A Large-Scale Real-World Amodal Tracking Benchmark
Traditional tracking (top) and Amodal tracking (bottom).
To address the scarcity of amodal data, we introduce the TAO-Amodal benchmark, featuring 880 diverse categories in thousands of video sequences. Our dataset includes amodal and modal bounding boxes for visible and occluded objects, including objects that are partially out-of-frame.
Our dataset augments the TAO dataset with amodal bounding box annotations for 17k fully invisible, out-of-frame, and occluded objects across 880 categories. Note that this implies TAO-Amodal also includes modal segmentation masks.
Amodal Expander
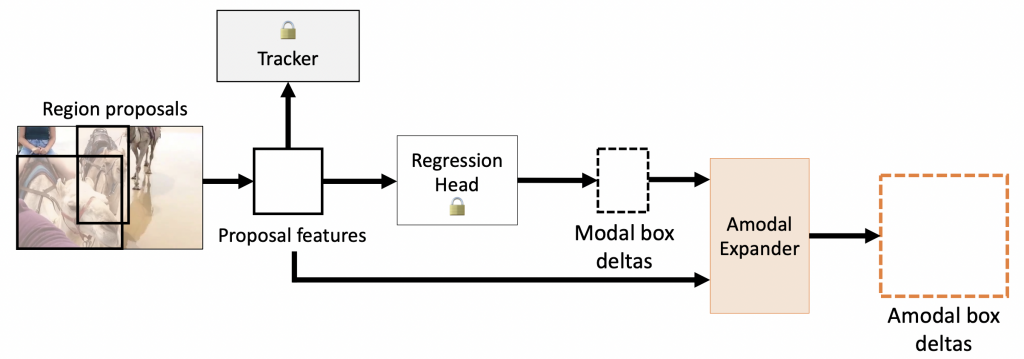
Our Amodal Expander serves as a plug-in module that can amodalize
any existing detector or tracker with limited (amodal) training data. Here we provide qualitative results of both modal (top) and amodal (bottom) predictions from amodal expander.
PasteNOcclude
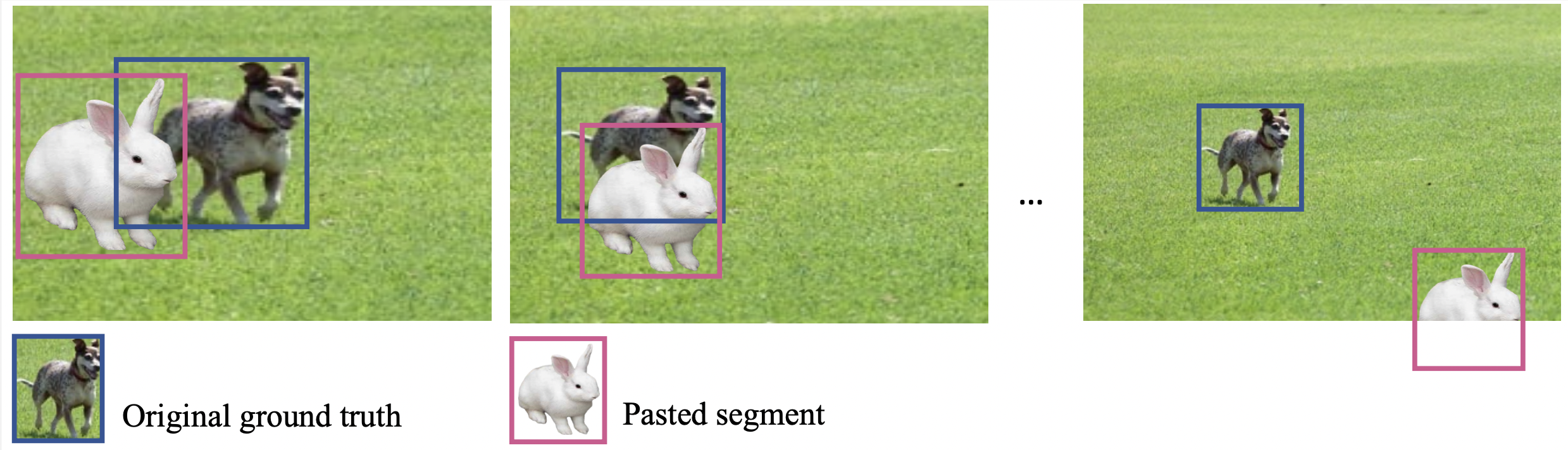
PnO allows us to manually simulate occlusion scenarios and out-of-frame scenarios. We randomly choose 1 to 7 segments from a collection sourced from LVIS and COCO for pasting. For each inserted segment, we randomly determine the object’s size and position in the first and last frames. The size and location of the segment in intermediate frames are then generated through linear interpolation.