For comparison, below we report the performance of the model on our full available training data of our synthetic data.
MIoU: 71.5
Top 3 classes:
Hips: 90.94,
L. thigh: 88.98,
R. thigh: 88.81
Worst 3 classes:
L. fingers: 22.33,
R. fingers: 22.77,
R. wrist: 48.01
We first started by sampling images using a keypoint segmentation consistency acquisition function, most sampled actions involve occlusions which are expected. However, a big problem with keypoint-based strategies is that segmentations are very coarse in earlier stages with fewer data, and thus key points could be off even for simple poses. On the contrary, most mistakes are fine-grained in the later stages, and key points don’t give enough signal.
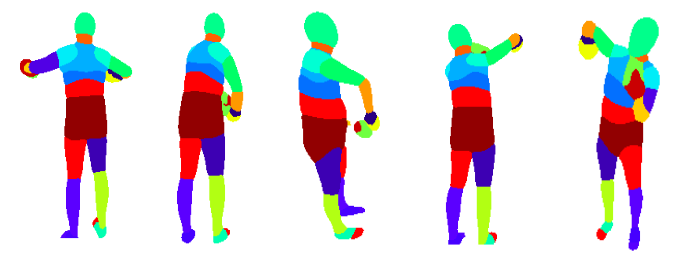
Next, we take a deeper analysis of our proposed multi-view consistency-based hard example mining strategy.
The example below shows a close-view of multi-view consistency across different views warped into the original point of view.
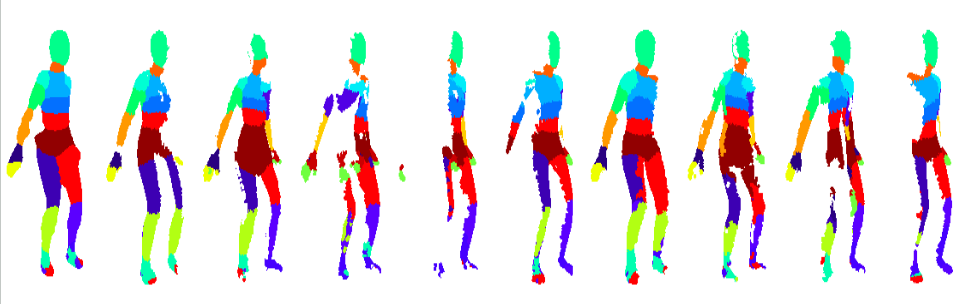
Results on data at Meta:
First, we adapted our multi-view sampling approach trained on our synthetic data to Meta’s data and demonstrated promising results. Our models without any fine-tuning perform well on Meta’s data since we tried to maintain domain similarity with backgrounds from the CMU panoptic dataset while generating our dataset.
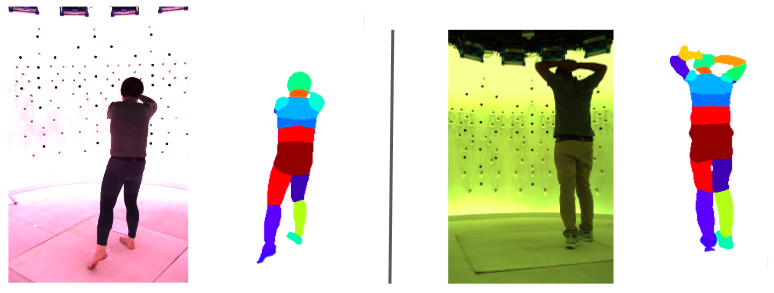
Next, we run our complete AL pipeline on internal datasets at Meta. Using 35% of the training data, we were able to achieve ~90% of full training data MIoU and our method clearly outperforms the baselines with the trend continuing as the percentage of used data increases. Quantitative results are shown below.
Finally, we have also explored the use of pseudo labels from easy poses and re-weighting strategies and the early results look promising. These methods will be further tested at Meta over the coming weeks before being shipped into production. In conclusion, in this work, we have shown how multi-view cues can be used to improve the label efficiency of models.