Part segmentation can reduce the ambiguity of meshes used in further downstream tasks for AR/VR at Meta. Multi-view(MV) part segmentation faces challenges due to complexity and high labeling costs/time (can take up to 1 min/annotation). Common failure cases at Meta and in our current pipeline involve self-occlusions, crossings between hands and feet, and complex joint actions where boundaries are unsure (forearm/upper arm under complex elbow rotations). We aim to construct novel active learning (AL) approaches to identify hard examples, maintaining ~90-95% of possible accuracy while reducing costs (with only ~30-35% annotated data). By selectively querying informative samples, we plan to leverage MV information to accelerate learning, offering an efficient solution for MV part segmentation.
Examples of self-occlusions and complex actions:

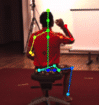
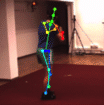
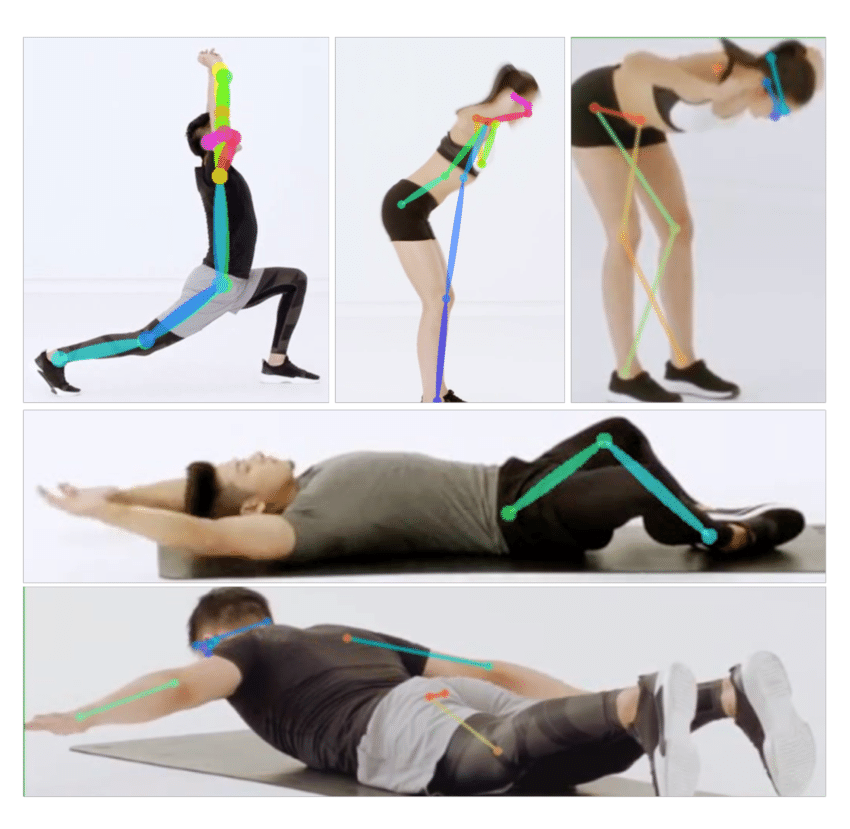
Contributions:
In this work, our contributions are listed below:
- Created a synthetic multi-view part segmentation dataset with 2.5M+ generated samples containing images, depths, and body part segmentation maps with 25 labels similar to SMPL annotations.
- A novel multi-view consistency-based sampling strategy for mining hard examples and using them for active learning.
- Demonstrated improvements on active learning on our generated dataset as well as Meta’s internal datasets.