Quantitative Results

We evaluate our automatic hitting angle estimation algorithm on three different datasets.
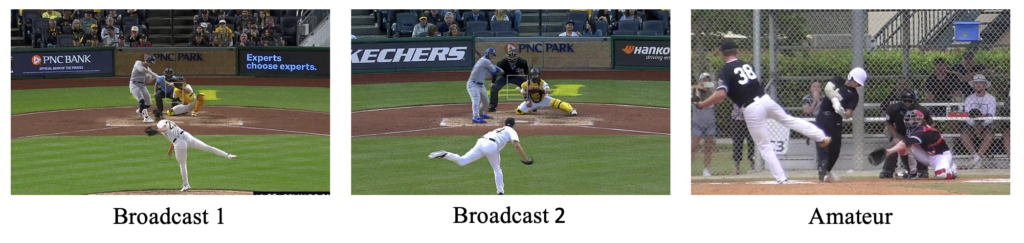
Broadcast Video Dataset 1: In the first dataset, where the camera is positioned directly behind the pitcher with minimal view deviation, our native angle estimation achieved a promising mean angle error (MAE) of less than 4 degrees. Comparing the ‘Angle MAE’ column with the ‘Angle MAE (With GT Keyframe)’ column, we observed that the estimation error is very close to the error obtained using ground truth keyframes, demonstrating the accuracy of our keyframe detection module.
Broadcast Video Dataset 2: In the second dataset, the camera is behind the pitcher but with a rotated view. By comparing the ‘Angle MAE’ with ‘Angle MAE (Corrected by VP)’ column, we found that the angle estimation error decreased significantly and became closer to the ‘Angle MAE (With GT Keyframe)’ value. This result verifies the effectiveness of our vanishing point-based angle correction algorithm.
Amateur Dataset: Finally, we tested our algorithm on a dataset of self-recorded amateur swing videos. This dataset exhibits substantial variation in viewpoints, backgrounds, and video quality. Moreover, due to the absence of professional stadium conditions, we lack the ground truth strike-zone hitting angle. Thus, we could only measure the 2D angle estimation MAE. The results showed an estimation error within 4 degrees, demonstrating that our algorithm is robust to diverse video qualities and viewpoints
Qualitative Results
Detection Results on Video Clip
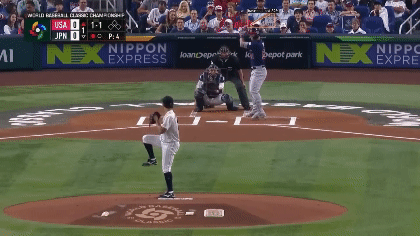
Example Keyframe and Angle Estimation
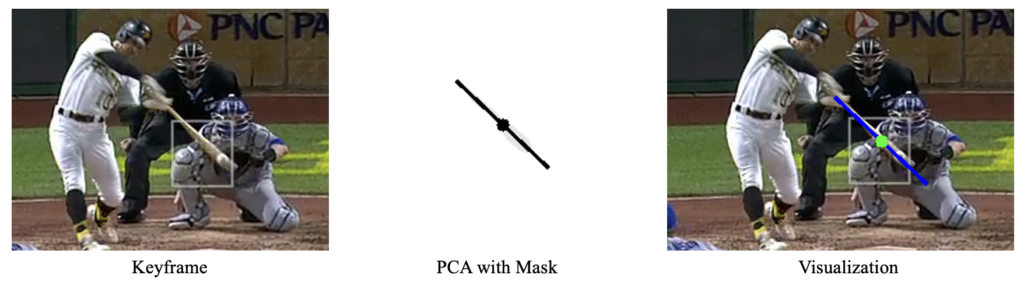