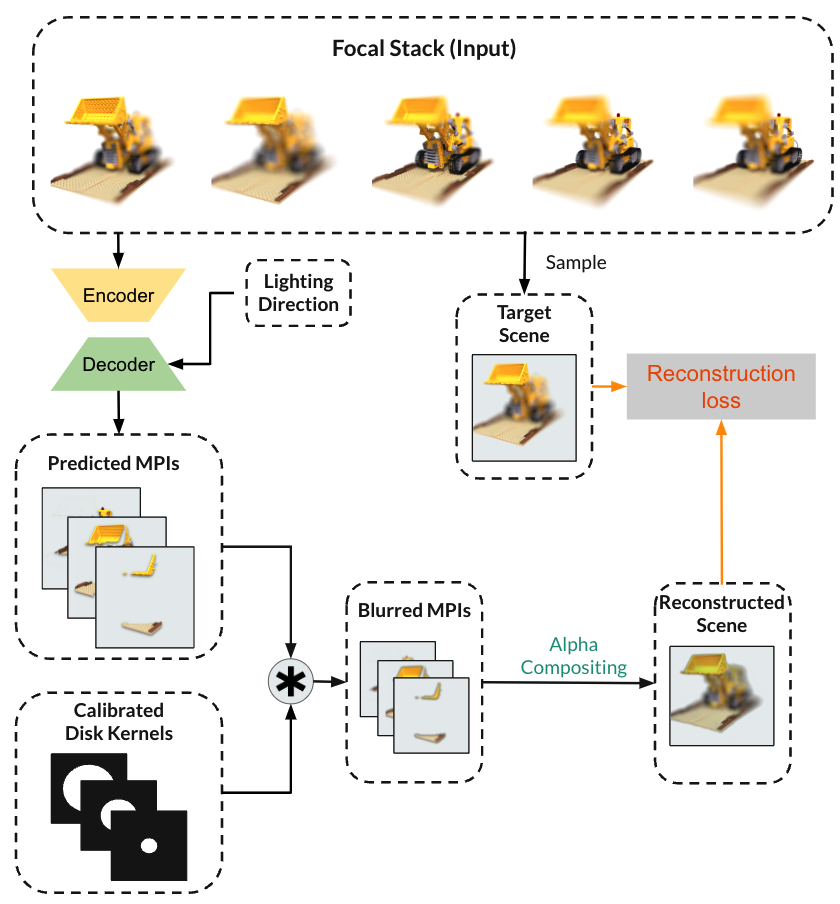
Here we describe each component of our pipeline for estimating depth from focal stack.
Multiplane Image (MPI)
We represent the scene with Multiplane Image, which is a set of fronto-parallel planes at different predefined depths of the scene. Each plane contains RGB value and alpha or transparency value at a specific pixel location and depth layer. RGB images could be rendered with alpha compositing, and disparity map can be generated by taking expected value of the disparity map.
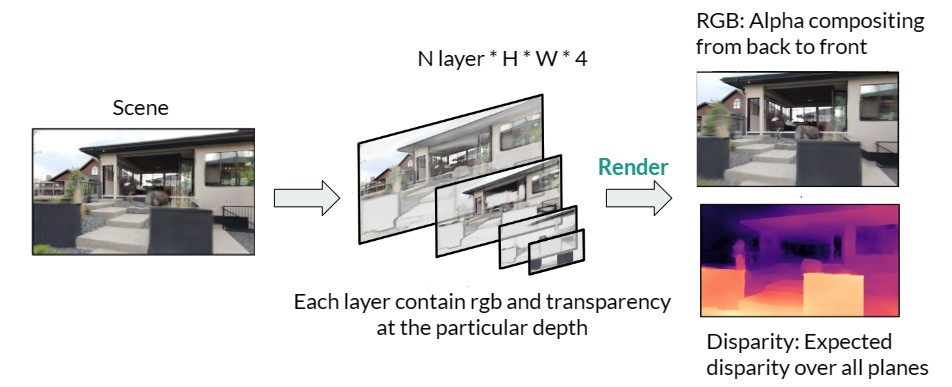
We chose the MPI representation due to its fast-rendering speed, known depth value of each layer and ability to work with patch-based losses such as GAN loss, Perceptual or SSIM losses.
Apply Defocus effect to MPI
To simulate defocus effect with MPI representation and reconstruct each image of a focal stack, we blur different parts of the scene with different amount of blur based on the difference between focal distance and depth at each layer of MPI. Specifically, we apply disk kernel with different diameter to each layer of the MPI.
Based on the Thin Lens Model, given camera parameter aperture and focal length, and focal distance which vary among images from the focal stack, diameter of defocus is at a particular scene depth:
aperture * |focal distance – depth| * focal length / (focal distance-focal length) / depth
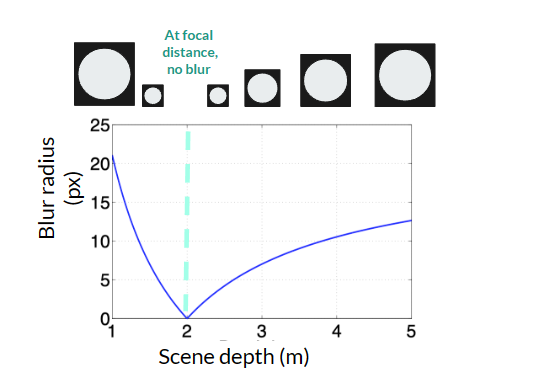
The above figure describes the relationship between blur amount and depth under a projective camera model. In our experiment, we are using an orthographic camera to capture the focal stack. With such a camera, the relationship between blur radius and scene depth is linear
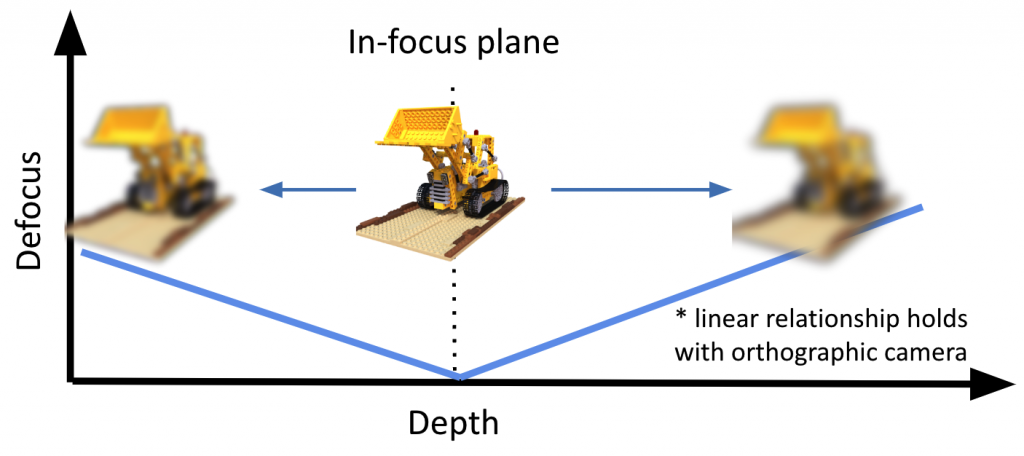
After convolving disk kernel of appropriate radius with each MPI layer, we perform alpha compositing of the layers to get the reconstruction. The reconstruction process is illustrated below:
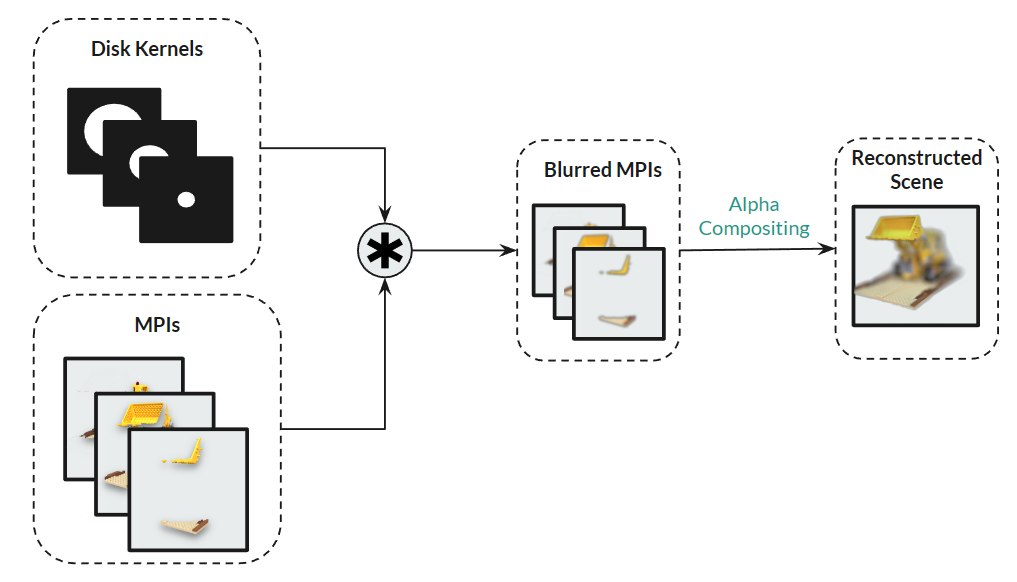
Optimization of MPIs
We compare the reconstructed image from the focal stack and the ground truth and optimize for the RGB and alpha values stored in each layer of the MPI through gradient descent by applying gradient of the loss with respect to the RGB and alpha values.
The loss functions we use include the following:
- RGB L1 reconstruction loss: enforces reconstructed image to have the same RGB value as the ground truth, L1 is used to increase sharpness
- Edge-aware smoothness loss: Defocus cues are inherently limited to surfaces with little or no texture. The edge-aware smoothness loss encourages that depth remains smooth with a textureless surface.