We present results on 3D face reconstruction achieved by our shape model and compare it with state-of-the-art models in texture and shape prediction. Once our end-to-end pipeline is trained and can produce albedo and mesh for a latent code z, it offers a lot of opportunities to leverage latent space manipulation applications in 3D. Since our whole pipeline preserves the latent space of StyleGAN, we can use latent space navigation to achieve expression manipulation in 3D. We can also perform text-based 3D face editing using contrastive Language-Image Pretraining using the StyleCLIP model.
Below are some of the results achieved by our proposed pipeline:
Figure 1: Applications of our proposed pipeline in real-world
Results – 3D Face Reconstruction
Quantitative comparison with SOTA in Mesh Prediction
Table 1 depicts the comparison of our model with the current SOTA methods, including DECA and MICA. Figure 2 illustrates the visual comparison among these methods. Our model outperforms the DECA model by achieving a 23\% better median error in coarse mesh and a 20% better median error in the detailed mesh. Our approach can reconstruct realistic-looking rendered faces, and model accurate head shapes, especially for faces with big heads.
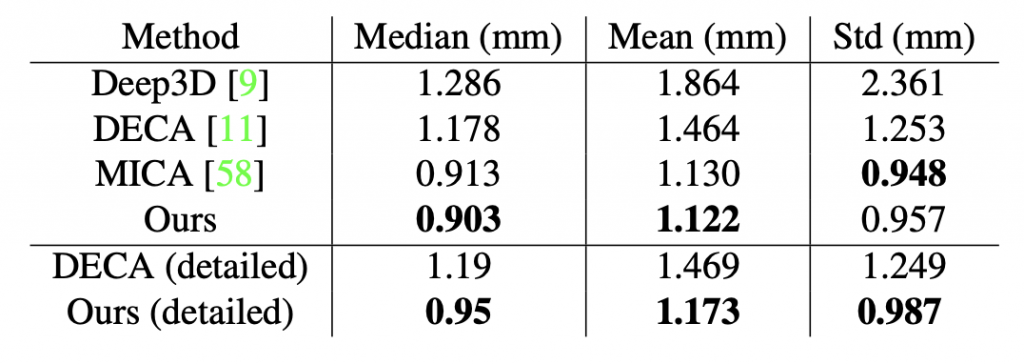
Table 1: Reconstruction error on the validation set (NoW)
Qualitative Results for Mesh Prediction
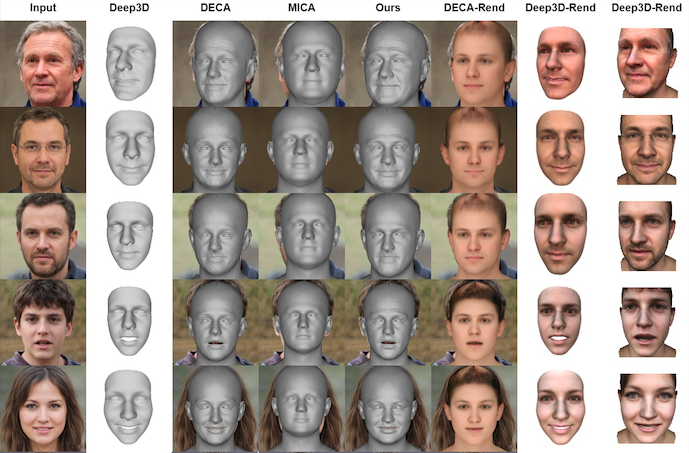
Quantitative comparison with SOTA in Texture Prediction
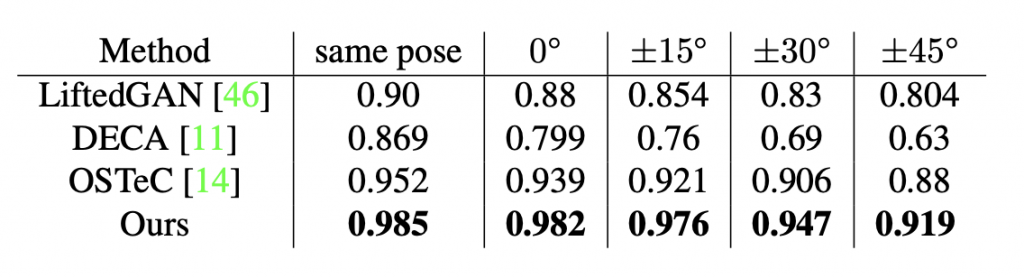
image, as well as 3D faces rendered in various poses.
Qualitative Results for Texture Prediction
We evaluate the quality of texture by comparing qualitatively to a recently proposed method, OsTeC. We also quantitatively compare texture and rendered faces by rendering them on a mesh with other methods, including LiftedGAN, DECA and OsTeC.
Fig. 3 shows the visual comparison between OSTeC and our model. OSTeC uses latent optimization-based GAN inversion which adds random artifacts in the generated image like the beard appearing on the chin as can be seen in the second row of Fig. 3. Furthermore, this leads to inconsistency while stitching textures across different poses.
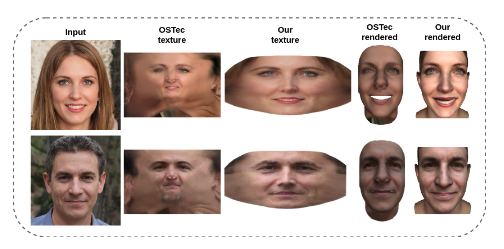
Results – Illumination Control via Lighting Model
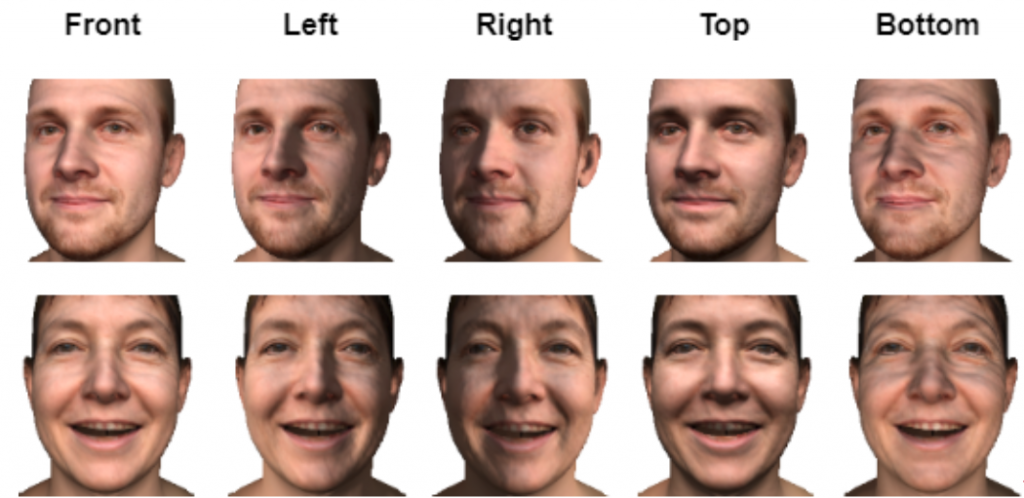
Results – Pose control on 3D meshes
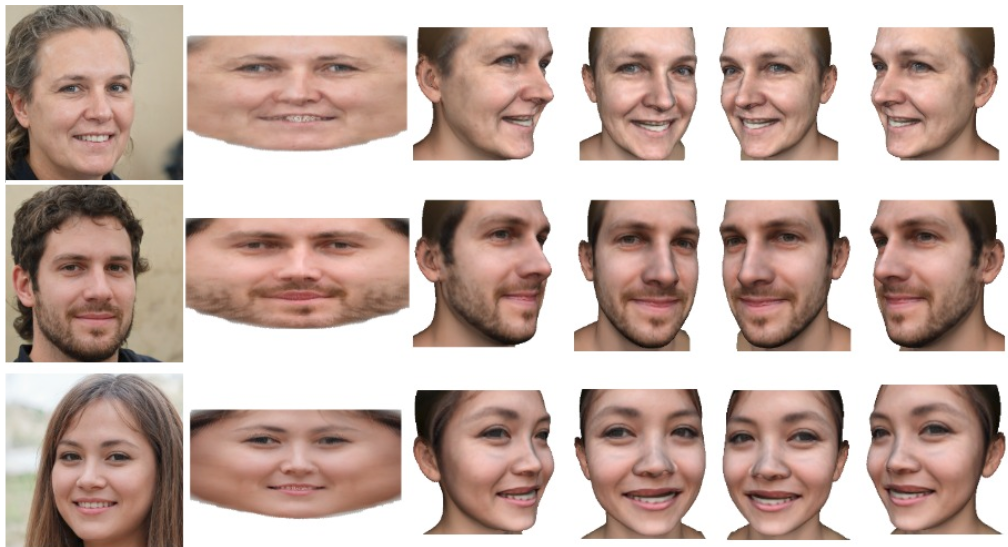
Results – Expression Control
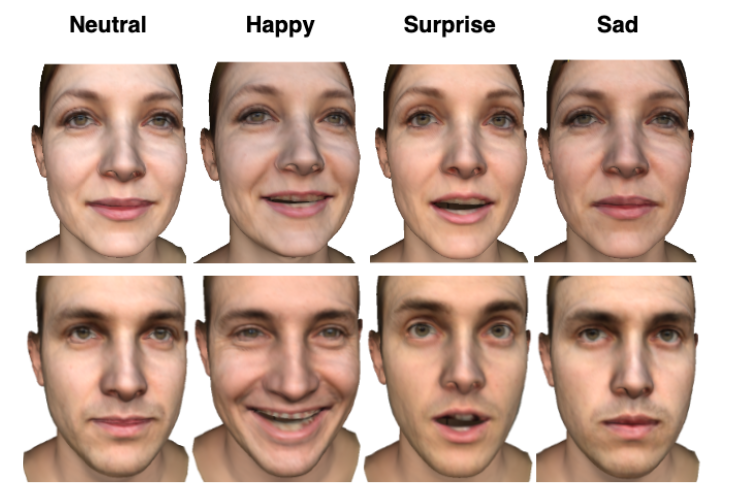
Figure 6: Generating different expressions by manipulating StyleGAN latent space
Results – Text-Based 3D Editing
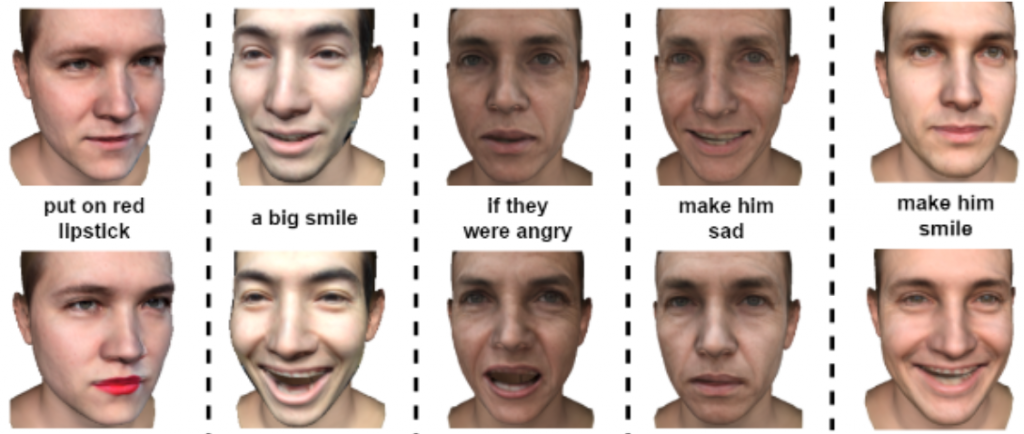