New dataset and pipeline
To review, the challenges we face with Synthetic Dataset generation are as follows:
- It is very time-consuming to build a dataset.
- Multiview setting requires a lot of hacking to the game.
- Domain transfer would be a problem
With these challenges in mind, we pivoted to the TBD Pedestrian dataset by CMU, which is multi-view and provides camera matrices and 2D trajectory labels for every pedestrian in all 3 camera views. The dataset is time synchronized so we know the frame number, pedestrian ID, and position coordinates of every person across all views.
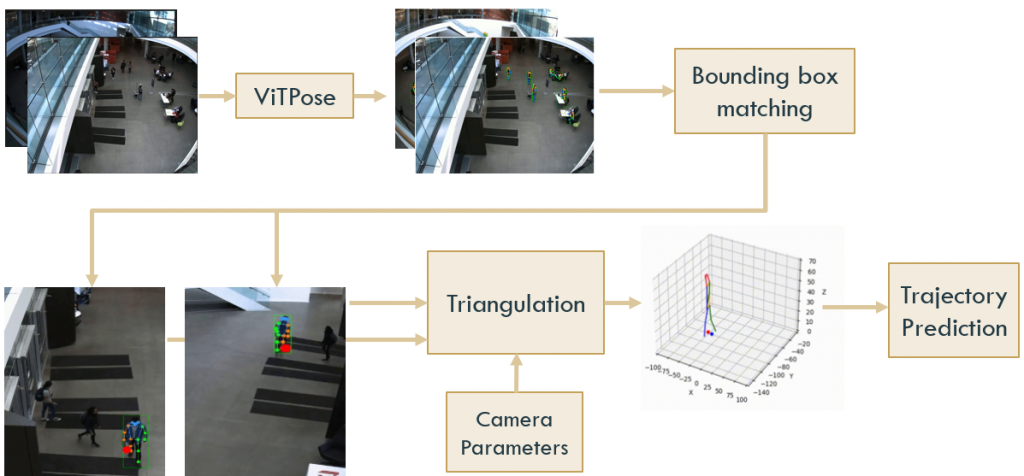
The pipeline we designed for 3D trajectory prediction is as follows:
- Perform 2D pose estimation with ViTPose on all 3 camera views
- Use a Greedy approach to match the bounding boxes of each pedestrian across at least 2 camera views.
- Create a dataset of 2D pose sequences for each pedestrian across 2 camera views.
- Perform triangulation using the camera matrices and the 2D pose sequences to obtain the 3D pose ground truth for each pedestrian
- Perform trajectory forecasting to predict the 3D trajectories of each pedestrian
(I) 2D Pose Estimation with ViTPose
Results of ViTPose on TBD Dataset
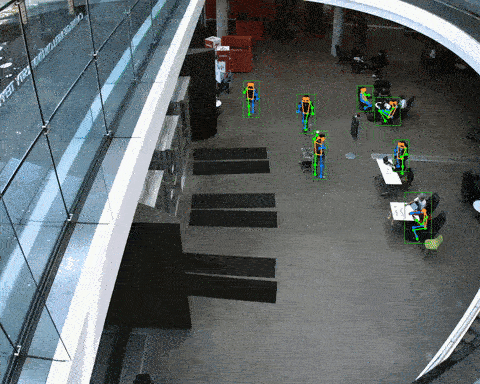
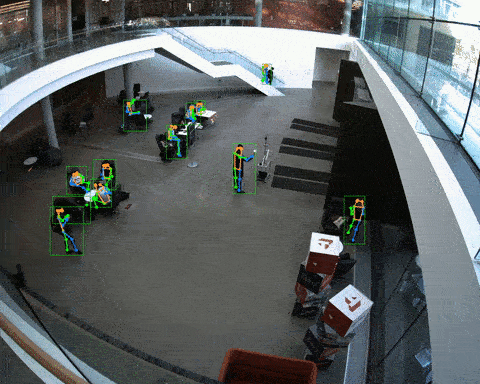
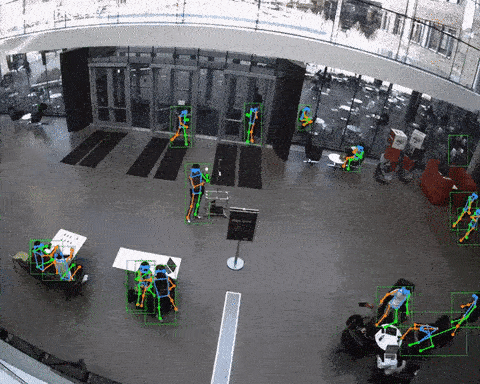
(II) Bounding Box Matching
Now that we have obtained the bounding boxes and 2D pose key points for each pedestrian, we need to match them across camera views. We need 2D pose sequences for each pedestrian across camera views so that we can triangulate them to obtain 3D pose key points. Since 2 views are sufficient for triangulation, we perform bounding box matching for View 1 and View 2.
To match pedestrians across views, we use the following greedy approach:
- for each pedestrian with a trajectory label and pedestrian ID, for the first frame of that pedestrian ID, we find the closest bounding box to the trajectory point.
- for subsequent frames, we track that bounding box, by finding the bounding box with the highest IOU with the previous frame
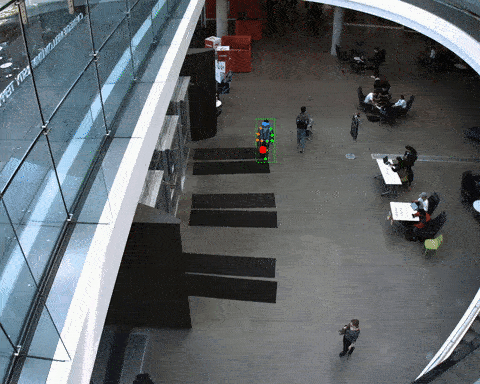
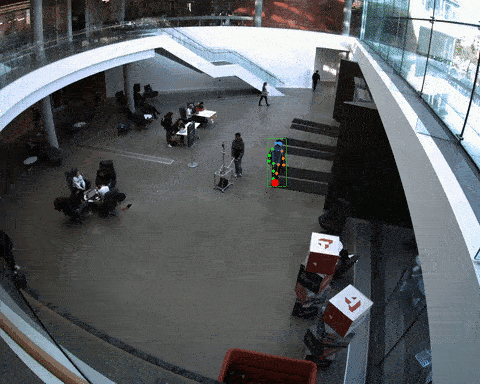
We now have a sequence of 2D pose trajectories for each pedestrian in both views, on which we can now perform triangulation to obtain 3D pose information.
(III) Triangulation
We use 2D pose sequences from view 1 and view 2, and the camera matrices for triangulation to obtain 3D ground truth pose sequences for each pedestrian.
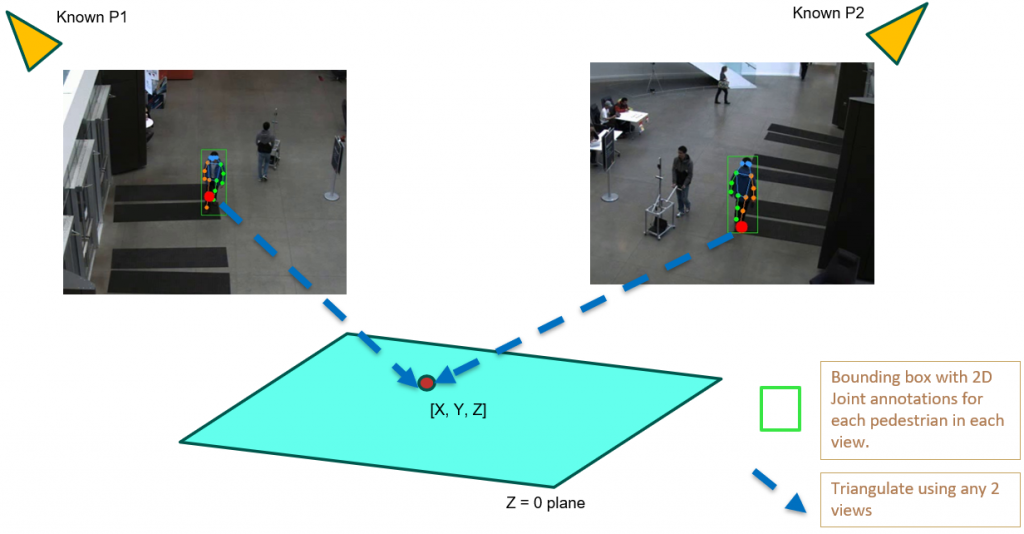
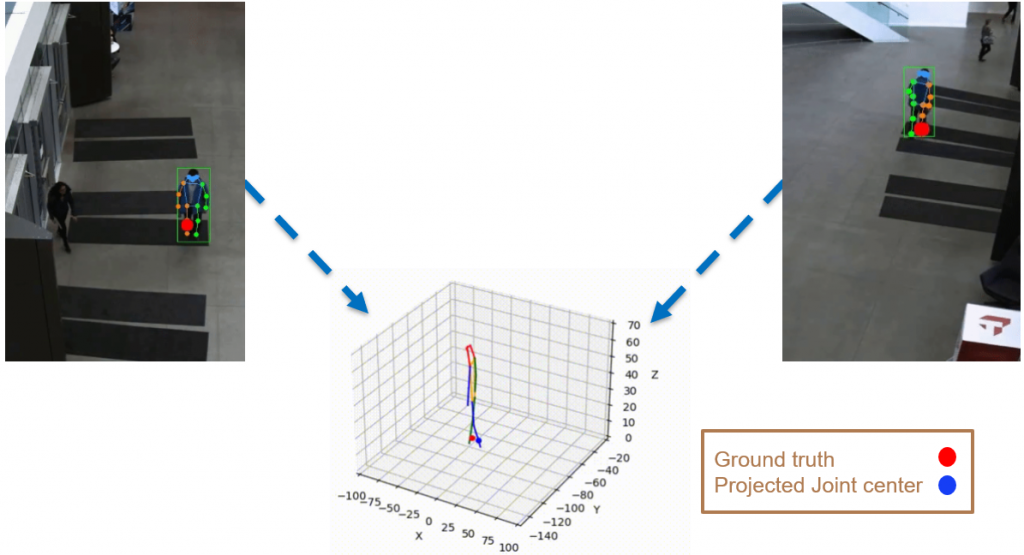
However, the triangulated 3D joints are noisy due to the downstream errors from both pose estimation and bounding box matching. Hence to obtain a smoother trajectory with less jitter we apply smoothening on skeleton gravity, limb length, and limb angles.
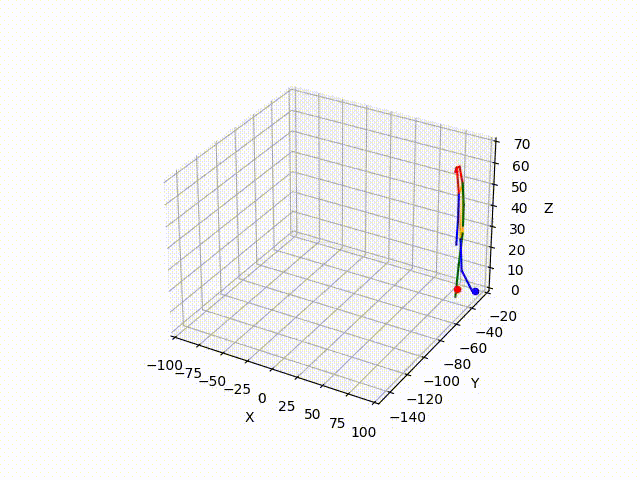
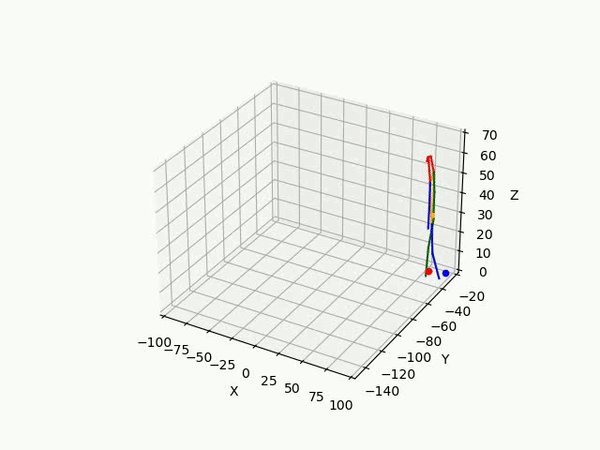
(IV) Trajectory Forecasting
We take 2 approaches to trajectory forecasting:
- Predicting 3D point trajectory
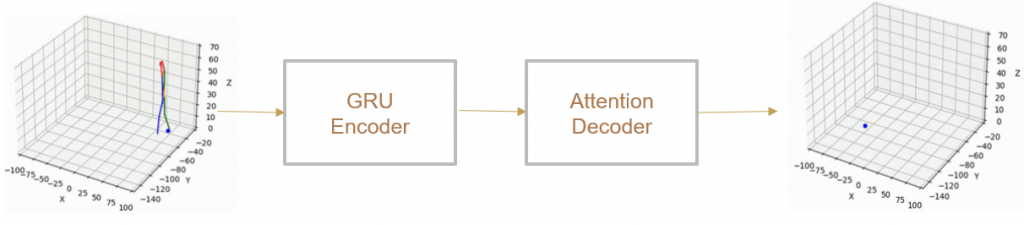
Result
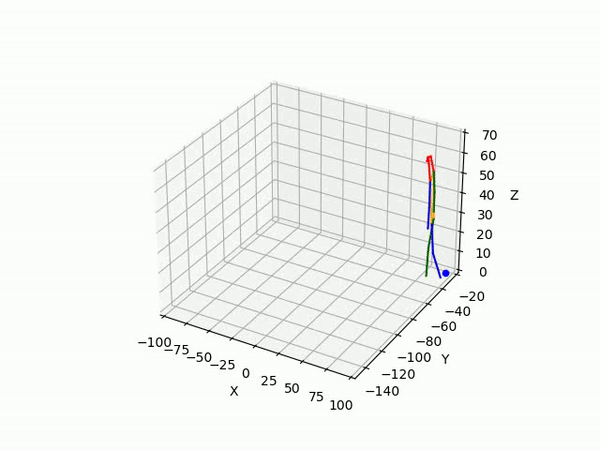
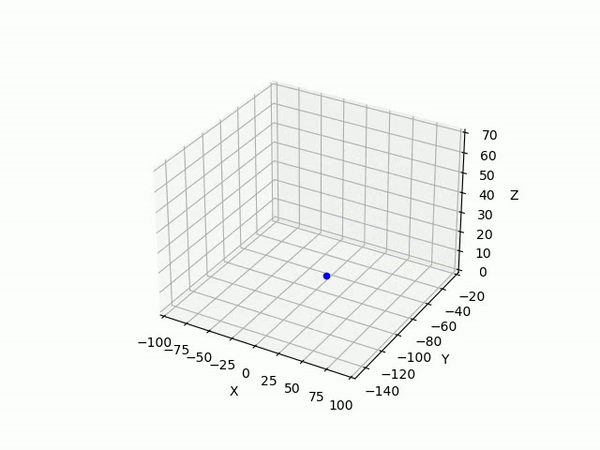
2. Predicting 3D skeleton and trajectory
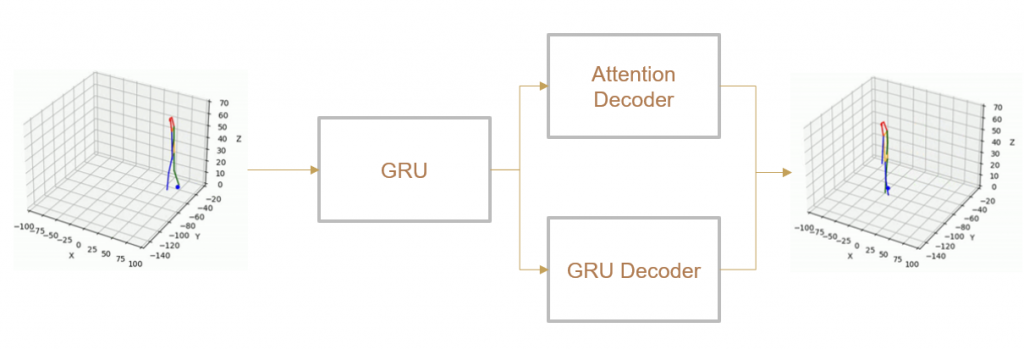
Result
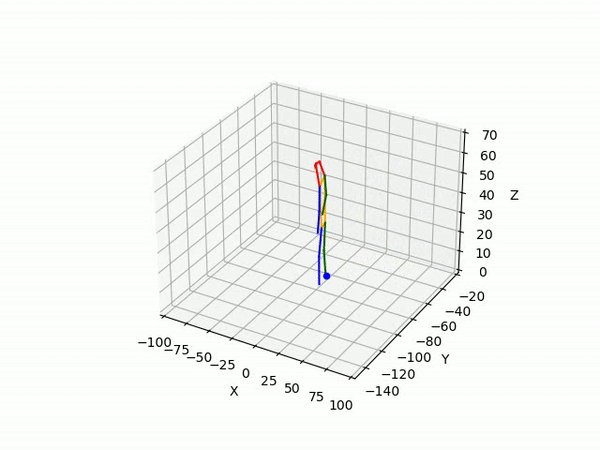
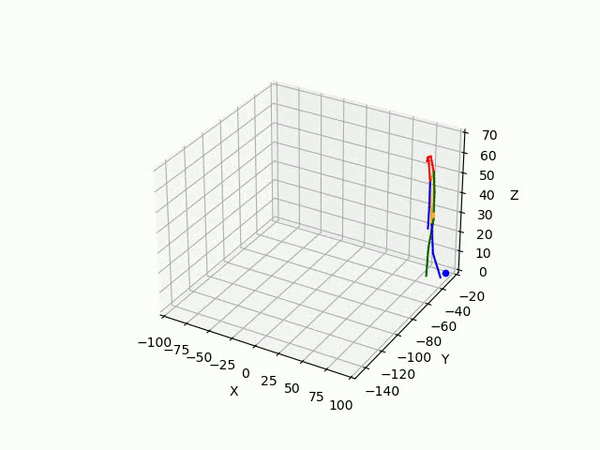