Qualitative Results
Qunatitative Results
We compare our method of text-to-cinemagraph, for generating cinemagraphs from text against 2 categories of baselines:
(i) image-to-cinemagraph: where we first generate image using Stable-Diffusion, and then generate cinemagraph from this image using prior works (Animating Landscape, Holynski et al. 2021, SLR-SFS)
(ii) text-to-cinemagraph: where we directly generate a video from text using prior methods (Text2Video-Zero, CogVideo, VideoCrafter) and then make them looping as a post-processing step
To evaluate the quality of our generated results, we perform human evaluation, where novice users on Amazon Mechanical Turk were given a text prompt asked to select which cinemagraphs between the one generated by our method and the baseline looks better. Each comparison with a baseline has recieved more than 800 annotations.
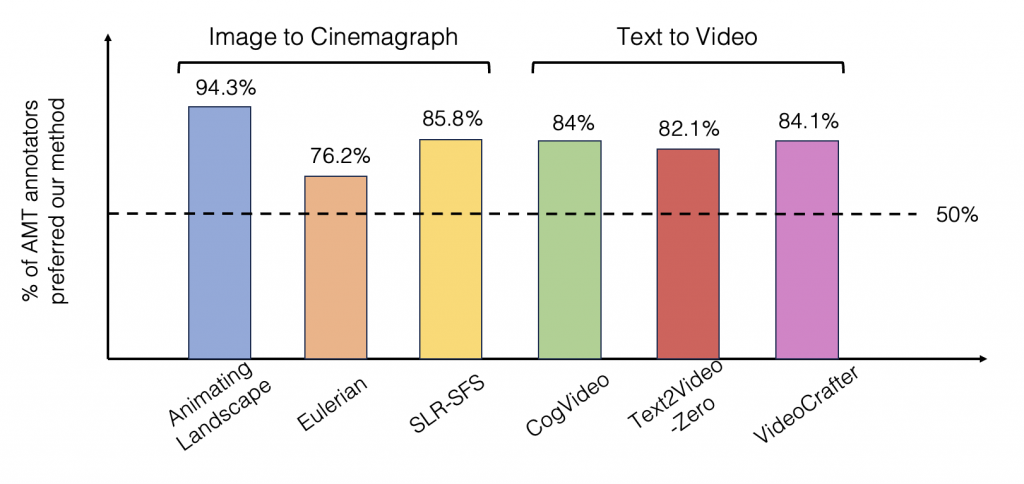
We show a plot of what percentage, of users prefer our cinemagraphs compared to the baseline. From the plot above, we find that compared to cinemagraphs generated by both category of baselines, users prefer ones generated by our method by a large margin.