We conduct experiments on two different domain adaptation benchmarks, i.e. Office-31[1] and Office-home[2], to show the superior performance of our methods. Since Office-31 is a relatively small dataset, we label 30 images in each active learning stage. As for Office-home, we label 1% of unlabeled data in each stage. First, we compare with the pure-score-based sampling strategies where we directly choose samples with the highest acquisition scores (best performance highlighted in bold). The result shows that our proposed methods consistently outperform entropy, a widely used uncertainty estimator. In addition, we also modify CLUE, which is a state-of-the-art method considering both uncertainty and diversity, based on our proposed score. The model surpasses CLUE ~1% on average top-1 accuracy in the early stages, which suggests that we need to consider both classification and domain alignment in the sampling process.
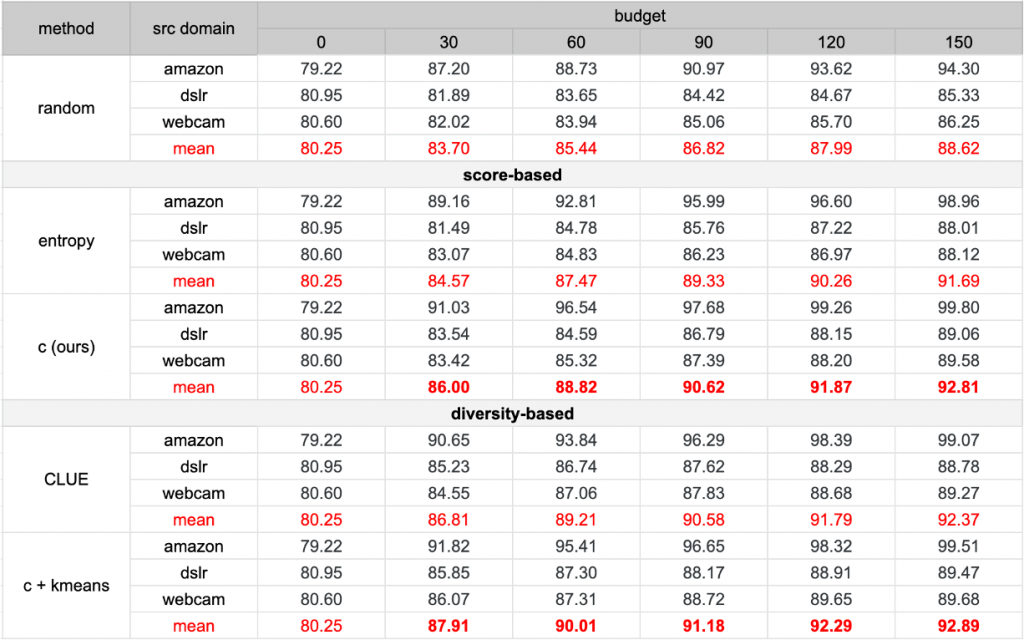
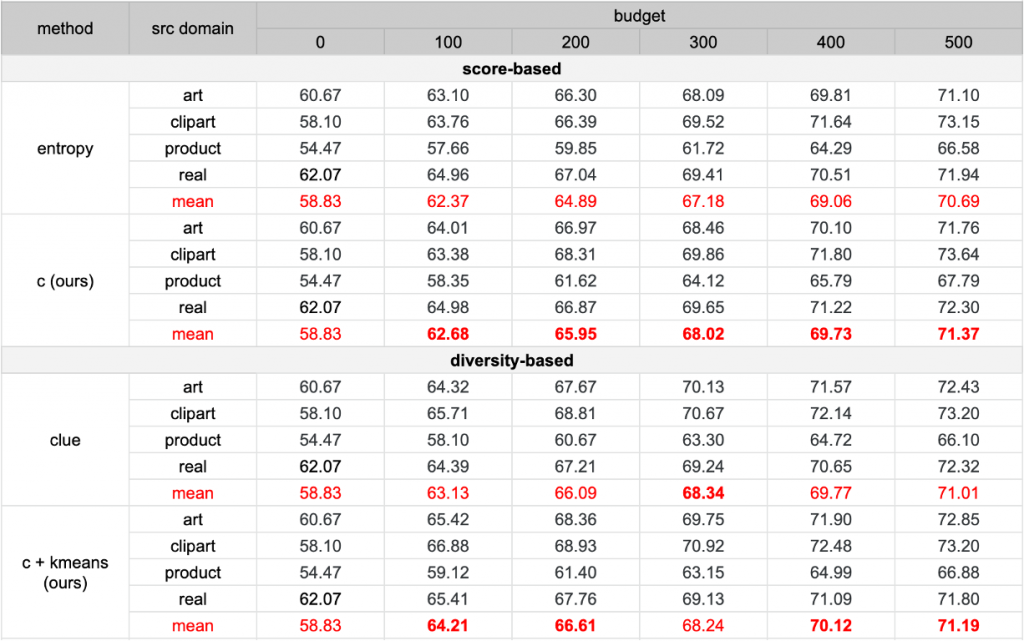
[1] Kate Saenko, Brian Kulis, Mario Fritz, and Trevor Darrell. Adapting visual category models to new domains. In ECCV, 2010.
[2] Hemanth Venkateswara, Jose Eusebio, Shayok Chakraborty, and Sethuraman Panchanathan. Deep hashing network for unsupervised domain adaptation. In CVPR, 2017.