Qualitative Results
In the following figure, we note that our Far nuScenes contain clean annotations at a distance and for scenes with cluttered objects (a). By comparing the predictions between CenterPoint (b) and FCOS3D (c), we observe the image-based FCOS3D contains higher quality predictions for Far3Det, which is not reflected in the standard evaluation. Our proposed fusion method AdaNMS (d) leverages their respective advantages and greatly improves detection of far-field objects. (Zoom in to see better.)
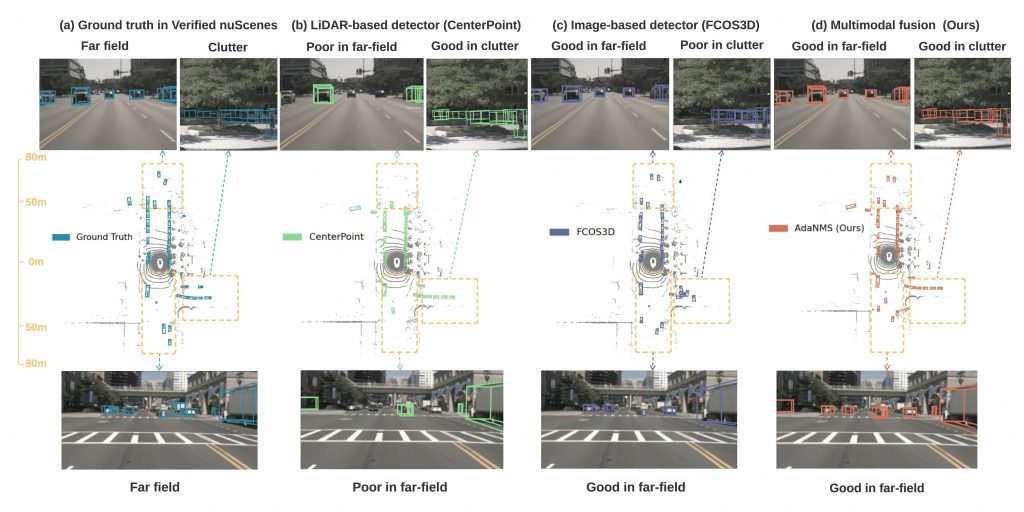
Quantitive Results
Here we show comparison of various evaluation protocols (3D mAP) on nuScenes for LiDAR-based CenterPoint and image-based FCOS3D. We calculate the AP metric using default thresholds of 0.5, 1, 2 and 4m, proposed adaptive linear threshold & quadratic threshold. We find low numbers for image-based method in 50-80m range using default metric that are not consistent with the visualization shown in Fig. 6. We posit this evaluation uses a too strict distance tolerance (e.g. 0.5m) in far field.
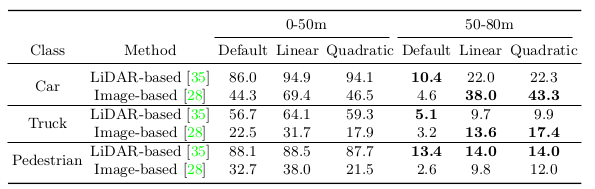
Far nuScenes version of Table 2. We observe similar trend as in but higher numbers. Recall that Far nuScenes is manually cleaned. We believe these improved results now realistically reflect the performance of 3D detectors for far-field detection.
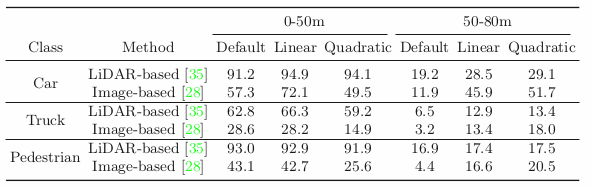
Finally, we show quantitative evaluation (3D mAP) on Far nuScenes under our proposed metrics based on linearly-adaptive distance thresholds. First, we notice that all LiDAR- based detectors perform well for the near field but suffer greatly in the far-field. Among these detectors, the VoxelNet-backbone CP(CenterPoint) significantly outperforms the rest. The image-based detector FOCS3D significantly outperforms CP for far-field. All fusion methods are able to take the “best of both worlds”, resulting in a significant gain for far-field (50-80m) accuracy. While being much simpler, our proposed methods NMS and AdaNMS fusion significantly improve upon more complicated baselines for all classes except Pedestrian, where a lower overlap threshold for NMS on far-field hurts the recall for cluttered scenes. *CP-VoxelNet is the same as CP appearing elsewhere in this paper. **Note that CLOCs3D is an extension of CLOCs. AdaNMS has two versions, one trained with MVP,
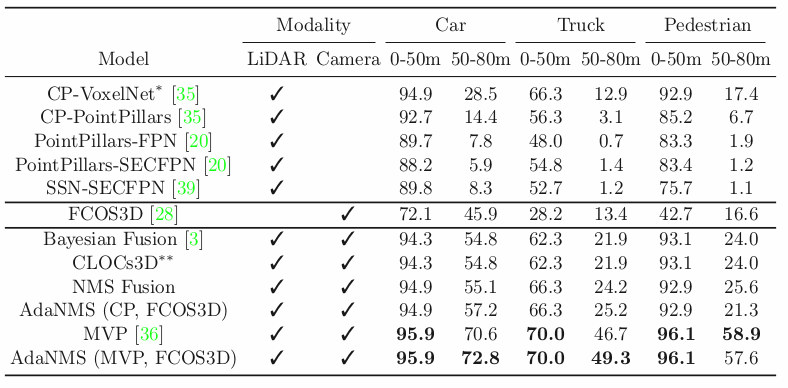