Multimodal Pedestrian Detection on KAIST
Quantitative Compomparison
Quantitative comparison on KAIST measured by LAMR↓ in percentage, on the two KAIST test-sets (old and new). We follow the literature that we evaluate in a “reasonable setting” [1], i.e., ignoring small or occluded persons. Our Bayesian Fusion approach (wtih bounding box fusion) is comparable in Table 1. We take reported numbers from [1] for most compared methods. Clearly, our Bayesian Fusion approach outperforms the prior methods by a large margin. Bolded numbers marks the best results
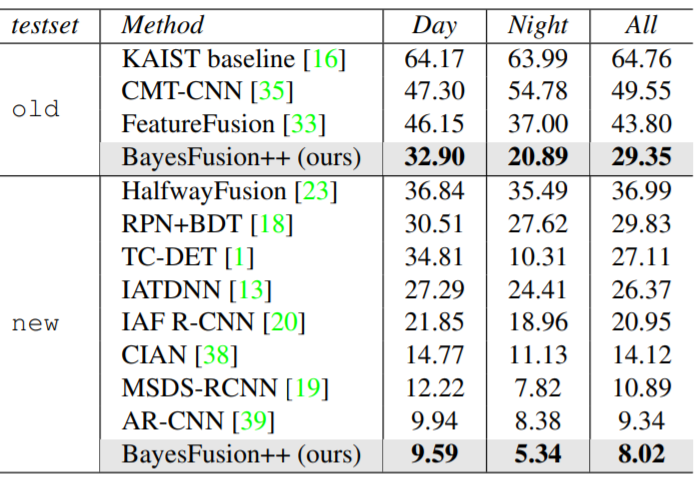
Ablation Study
Ablation study on KAIST new test-set under the “reasonable” setting, measured by percent LAMR↓. Please see text for a detailed discussion, but overall, we find our proposed BayesFusion approach to outperform all other variants, including end-toend learned approaches such as Early and MidFusion. Fig. 5 shows the corresponding MR-FPPI curves.
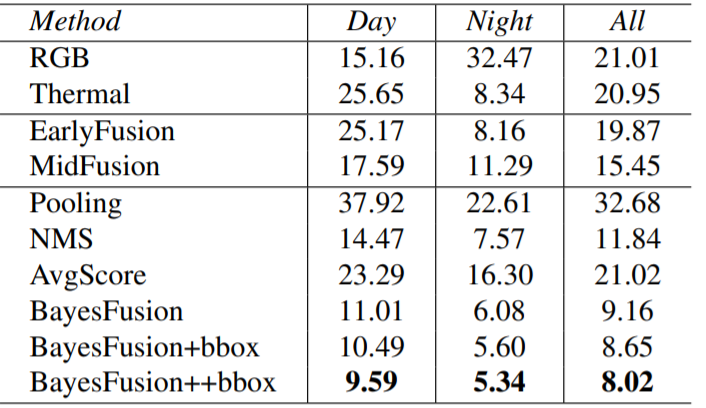
Quantitative Comparison
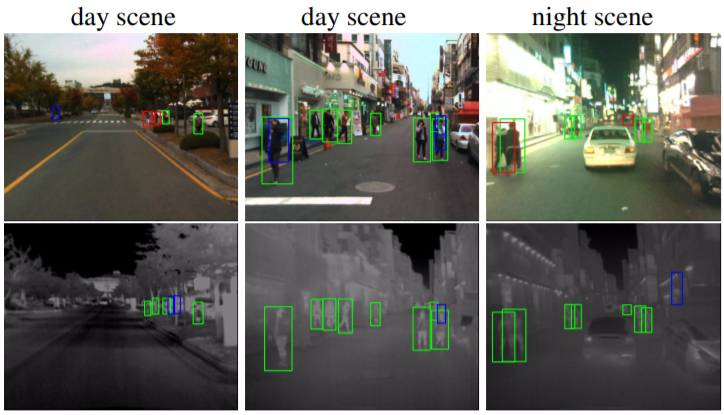
Multimodal Object Detection on FLIR
Quantitative comparison
Quantitative comparison on FLIR measured by AP↑ in percentage with IoU>0.5. Following the literature, we evaluate on the three categories annotated by FLIR. Perhaps surprisingly, end-to-end training on thermal images already outperforms all the prior methods, presumably because of better augmentations and a better pre-trained model (Faster-RCNN). Moreover, our fusion methods perform even better. Lastly, our Bayesian Fusion method performs the best. These results are comparable to Table 3.
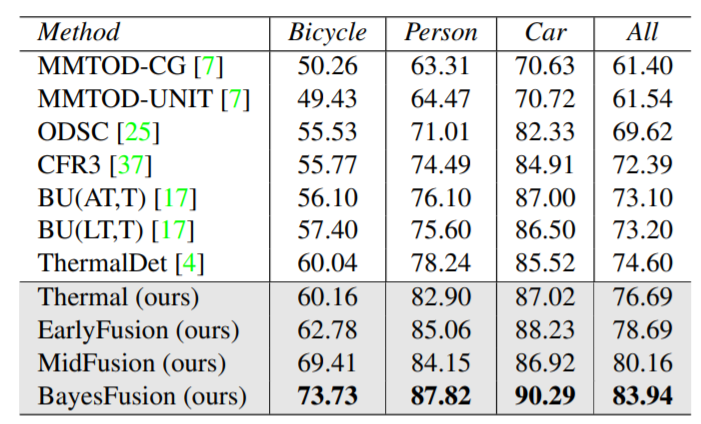
Ablation Study
Breakdown analysis on FLIR day/night scenes (AP↑ in percentage with IoU>0.5). As FLIR does not have day/night tags on the images, we manually annotate them for this analysis. Clearly, incorporating RGB by our learning-based fusion methods notably improves performance on both day and night scenes. We explore late-fusion with detection outputs from our three models: Thermal, Early and Mid. We find all AvgScore, NMS and BayesFusion lead to better performance than the learning-based MidFusion model. Especially, BayesFusion performs the best; using bounding box fusion (bbox) improves further
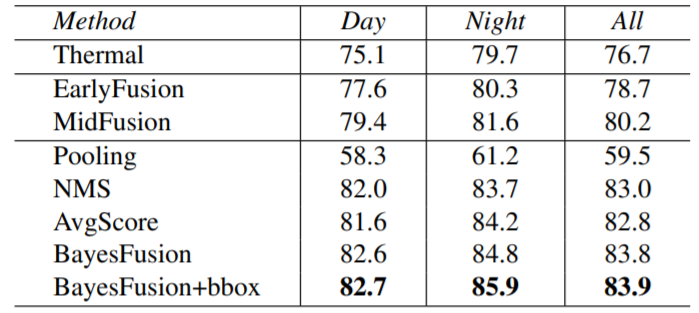
Quantitative Comparison
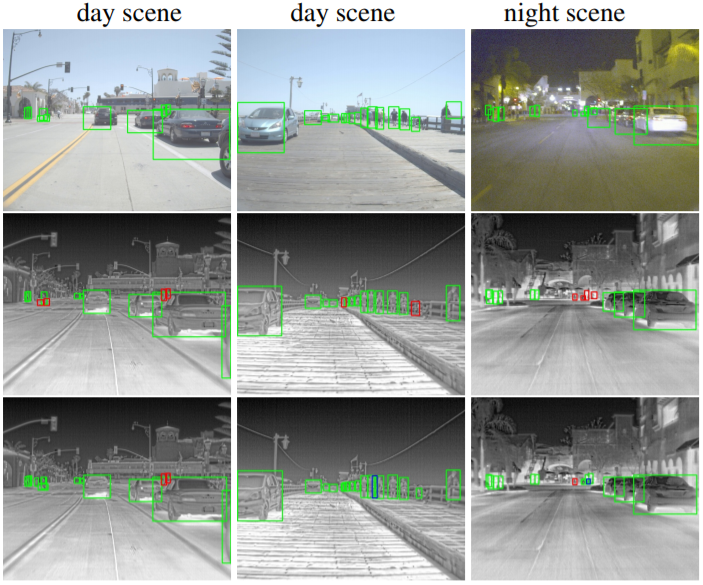