Abstract
This project explores the concept of reconstructing 4D scenes (3D space with time) using a single monocular camera during casual capture. Traditional 4D scene reconstruction methods often rely on specialized equipment, controlled environments, or multiple cameras. This project aims to overcome these limitations by developing a system that can reconstruct dynamic scenes using a single monocular camera during everyday activities, such as walking or hand-held recording.
Background
Reconstructing 4D scenes, has traditionally involved specialized equipment or controlled environments. Conventional methods may utilize:
– Multiple Cameras: Stereo rigs or camera arrays capture the scene from various viewpoints, enabling triangulation for accurate depth information.
– Depth Sensors: LiDAR or time-of-flight cameras directly measure depth at each image point, providing dense and accurate depth maps.
– Controlled Environments: Studios or motion capture stages utilize precisely calibrated camera setups and controlled lighting to simplify reconstruction.
While offering high-quality reconstructions, these techniques are impractical for capturing everyday scenes due to:
– Cost: Specialized equipment can be expensive and not readily available.
– Complexity: Setting up and calibrating multiple cameras or depth sensors can be time-consuming and require technical expertise.
– Limited Applicability: Controlled environments restrict the types of scenes that can be captured.
Method
1. Hybrid Scene Representation:
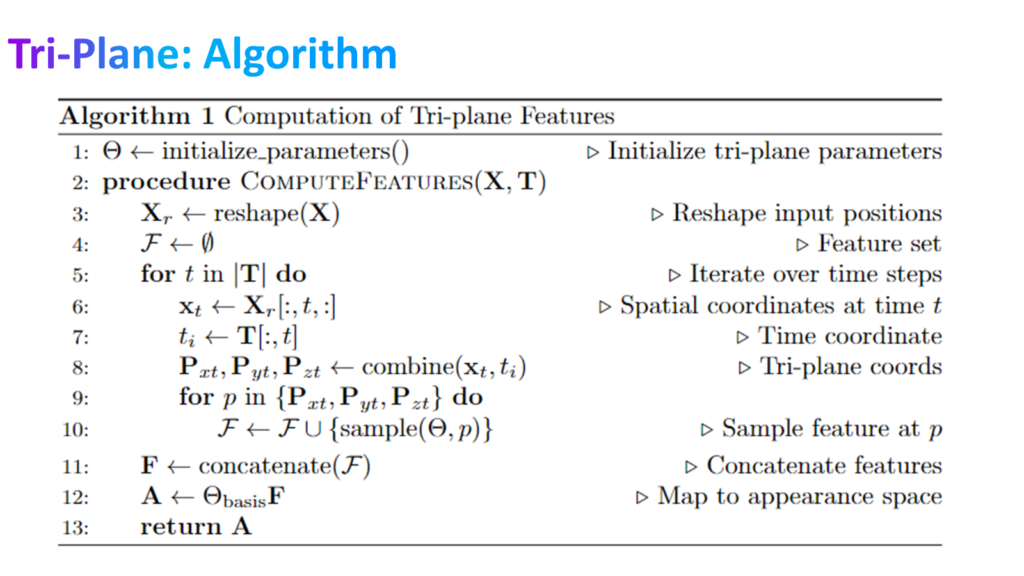
-The pipeline begins by capturing a dynamic scene using the monocular camera. A hybrid representation is then constructed, combining spatial and temporal information. This representation utilizes three 2D planes, forming a tri-plane approximation of the scene geometry.
-Additionally, a deformation field is incorporated to capture how objects within the scene move and change shape over time.
-This combined representation (tri-plane and deformation field) is fed into a Multi-Layer Perceptron (MLP).
-The MLP acts as a regressor, predicting the 3D coordinates (x’, y’, z’) of points within the scene.
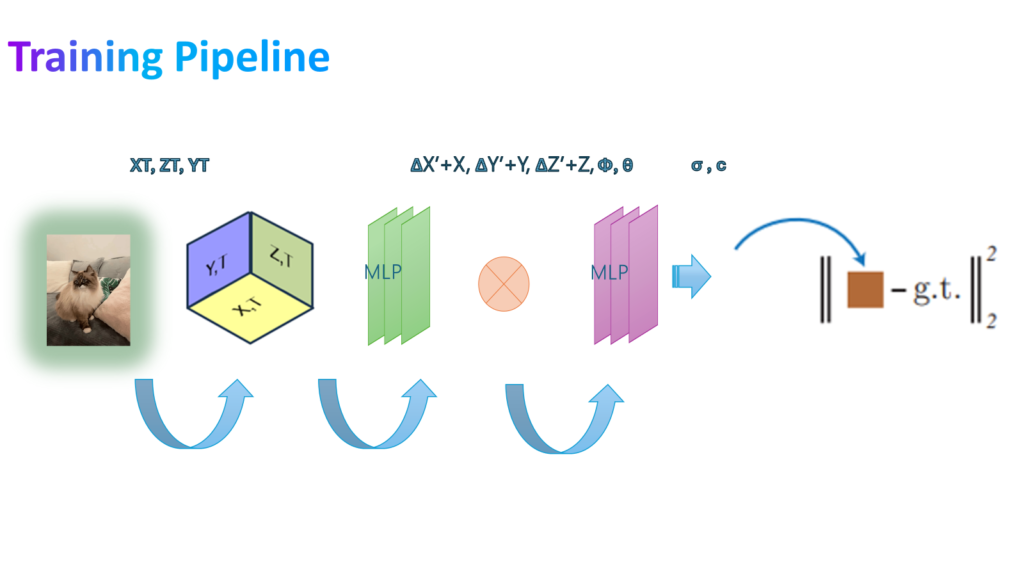
Results
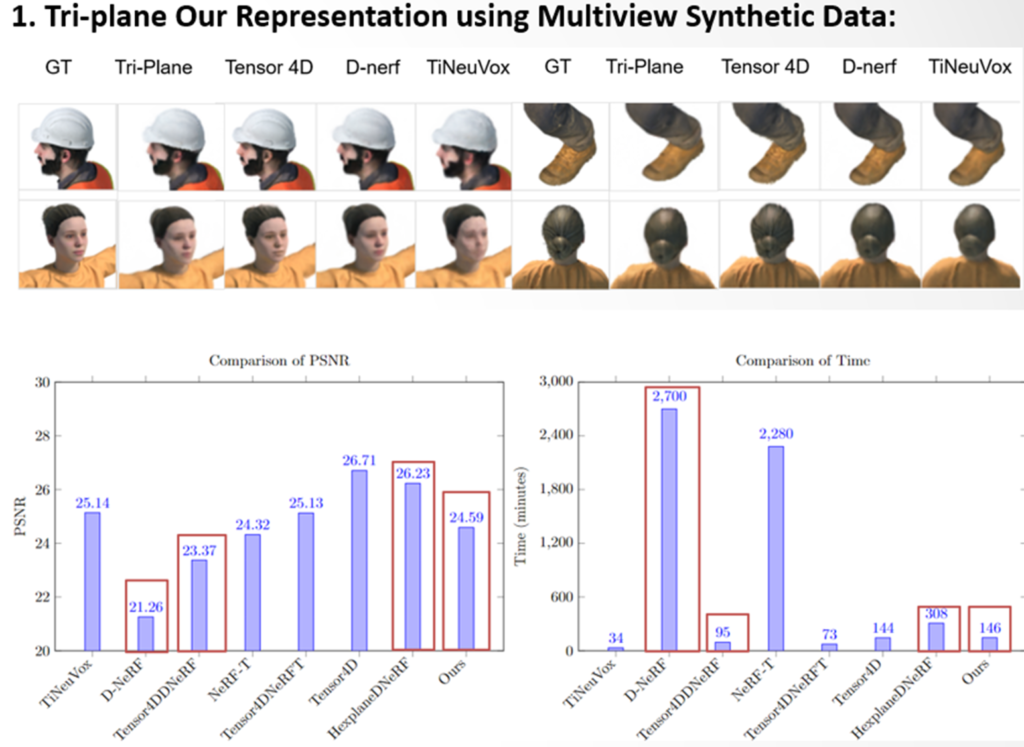
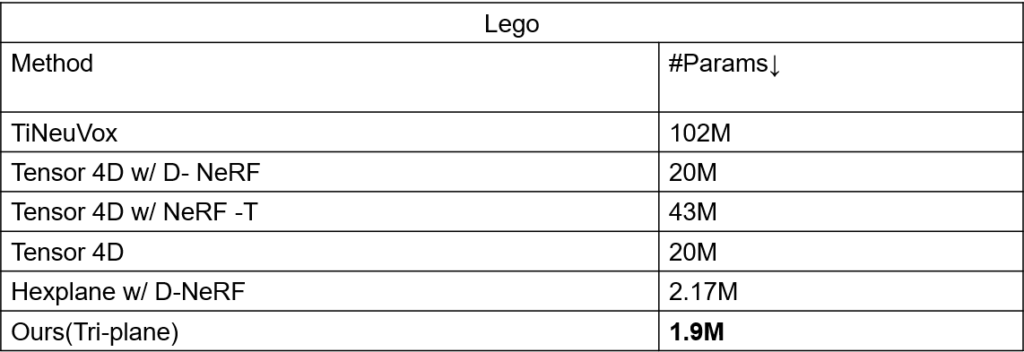
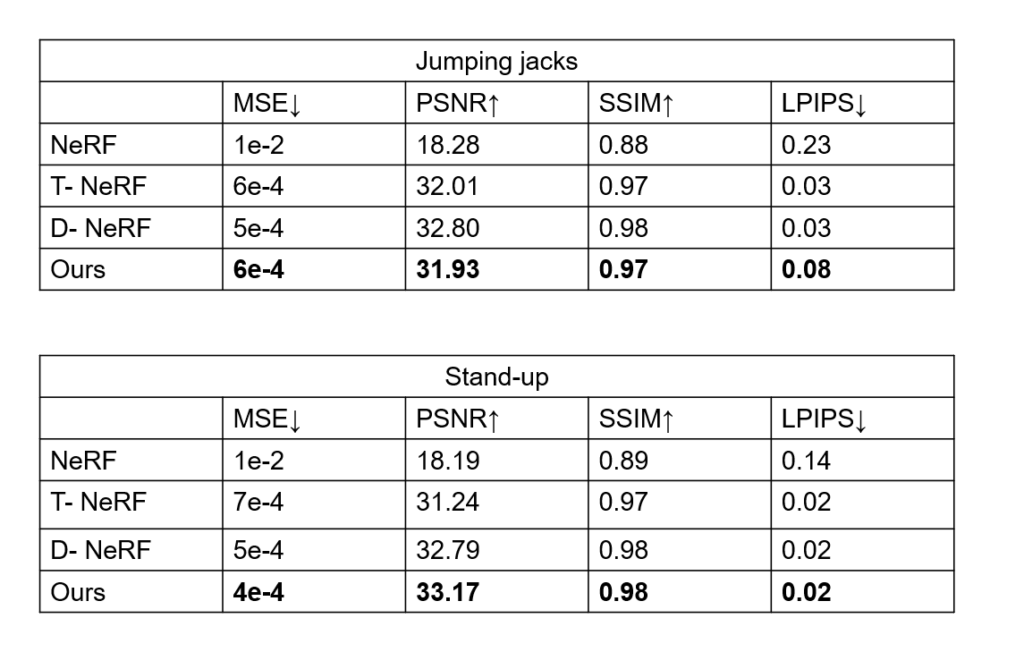
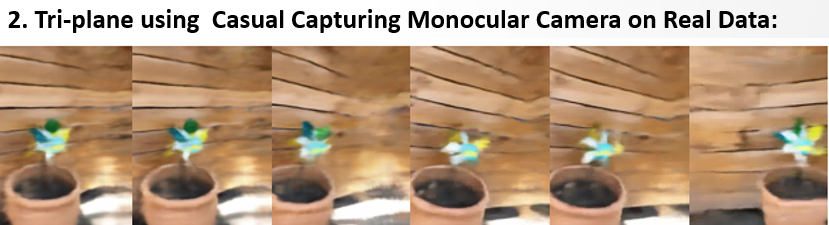
Presentation
Poster
Code
previous project
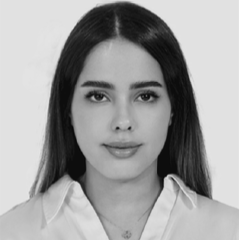
This work done by Asrar Alruwayqi, MSCV student. Under advice prof.Shubham Tulsiani.
bio
I am currently pursuing a Master’s degree in Computer Vision at Robotics Institute. Previously, I completed a Bachelor’s degree in Computer Science. Afterward, I worked as a Research Engineer at the National AI Center in Saudi Arabia, where I gained valuable experience and mentorship in the field. My ardor for computer vision is deeply rooted, with a special emphasis on 3D Vision, and computational geometry.
References and Credits :
Dynamic Novel-View Synthesis: A Reality Check.
D-NeRF: Neural Radiance Fields for Dynamic Scenes.
Tensor4D : Efficient Neural 4D Decomposition for High-fidelity Dynamic Reconstruction.
HexPlane: A Fast Representation for Dynamic Scenes.
Nerfies: Deformable Neural Radiance Fields.
NeRF: Representing Scenes as Neural Radiance Fields for View Synthesis.
TensoRF Tensorial Radiance Fields.