A classical problem in Computer Vision is to infer a 3D scene representation from a limited number of images. Another challenge is to obtain accurate camera and pose information for the captured images. Finally, real-world applications demand real-time inference of 3D representations and the ability to render novel views at interactive speeds.
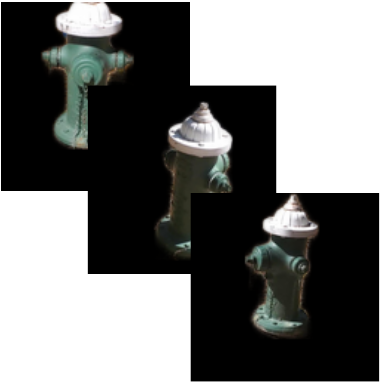
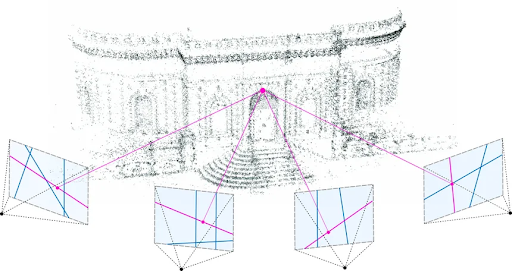
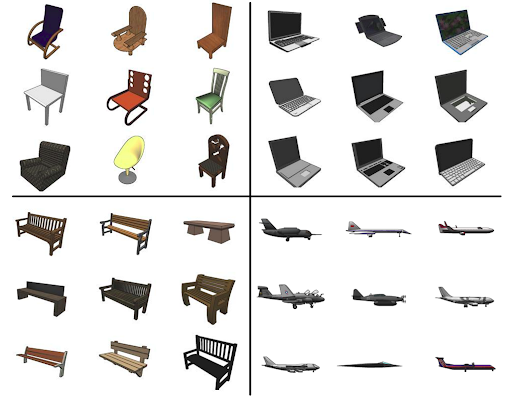
Sparse Input Views
Unposed
Generalizable to Unseen Categories
Such a technology has widespread applications in domains such as autonomous driving, robotics, real estate, AR, VR, e-commerce, fashion, and so on.
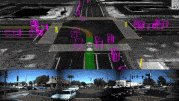
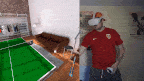
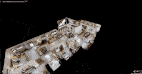
Autonomous Driving
Augmented Reality (AR)
Real Estate Virtual Tours
Thus, we propose a novel approach that, given a few RGB images of a previously unobserved scene with or without known poses, produces a 3D scene representation in a single feed-forward pass. Specifically, our method predicts 3D Gaussians that can be rendered from any novel view at interactive speeds.
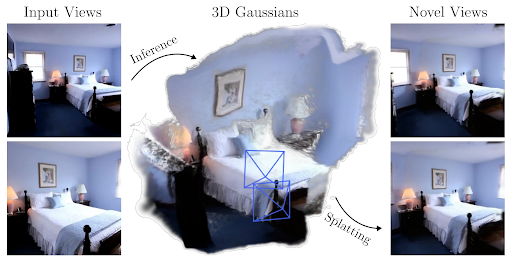